4 Steps Banks Can Take To Implement Next-Level AI-Driven AML
Banks are embracing artificial intelligence to help combat money laundering and financial crime at higher speed and with great accuracy.
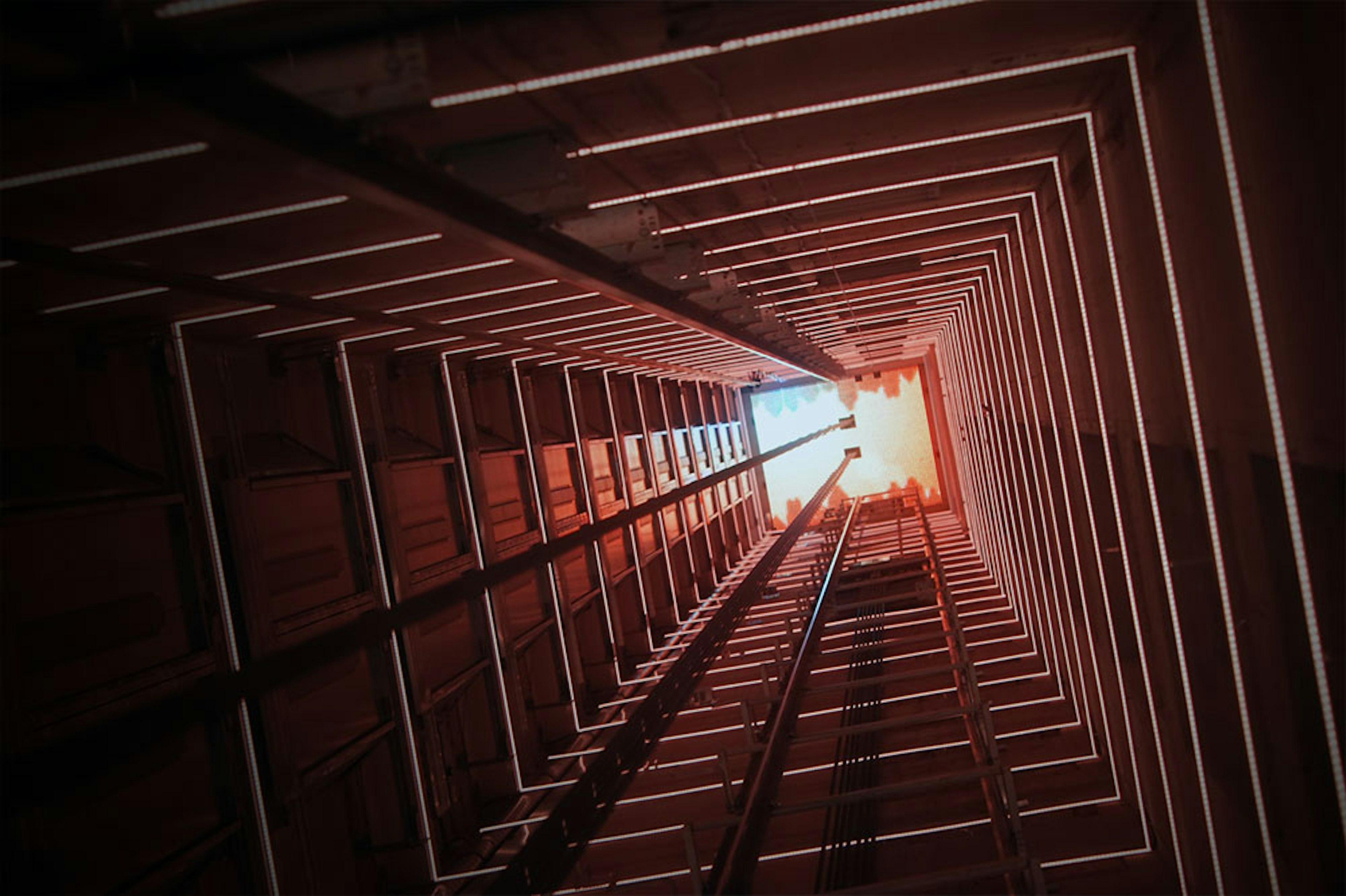
Artificial intelligence (AI) is a welcome addition to the anti-money laundering (AML) tool kit. Banks are using AI in the detection process for complex compliance activity, including wholesale and correspondent banking AML programs. But effective AI is wholly dependent on good data.
Here are four steps to maximize your AI efforts:
Build a strong data foundation
As the saying goes, if you put garbage in, you get garbage out. Organizations should endeavor to unify internal and external data at scale to build 360-degree views of entities (individuals, organizations, and locations) and surface a deeper understanding of connections, relationships, and patterns in data through Entity Resolution.
Leverage context in your data
Just like humans, AI relies on context to make well-informed decisions. Without context, even the most intelligent machine learning algorithms lack the accuracy needed for reliable deployment. By incorporating context-based techniques, banks can uncover relationships and patterns to reveal detailed interactions, such as transactional relationships or company affiliations.
Given that AI continuously learns from previous outcomes of money laundering investigations, it’s critical to strengthen the input data with relevant context to gain improved results. Contextual monitoring was born from the complex investigation space where enriched data and networks helped investigators find criminal activity. Taking this knowledge from the best investigators and converting that into detection algorithms created contextual monitoring.
For example, a contextual monitoring engine that uses information for all customers and inputs this into detection systems will be more accurate in its predictions; the ability to expand input from 30 to 300 relevant data inputs delivers an enormous uplift in performance.
Use various domain-specific models trained for specific outcomes
Your AI program can’t be a one-size-fits-all solution. Relying on one AI model, technique, or approach can lead to limitations in perspective, adaptability, and overall performance. For example, the methods and approaches used to build effective models in Retail AML, where there are many recorded outcomes, false positives, and most importantly, disclosures to the regulator (e.g., training data) might differ from approaches used in Trade Finance or Correspondent banking processes. In these cases, training data may be scarce. The scarcity requires a completely different approach and model that may rely on enhanced domain knowledge and subject matter expertise.
Prioritize explainability and transparency
Ensuring transparency and explainability of predictions and decisions is of utmost importance in AML and financial crime programs. As alerts are investigated by human analysts, it's critical to show them where the risk is and why the analytics triggered the alert.
A “white box” approach ensures you can meet regulatory and compliance requirements while customizing and tuning your models where needed. Both technical and non-technical teams should be empowered with the ability to easily explain the obtained results and validate the effectiveness of their models. Explainable outcomes will promote adoption and trust in your AI while also mitigating security, privacy, and ethics concerns.
“By replicating your best investigators’ approach across networked data, the AI will alert far more accurately and then provide the investigator team with a picture and wider, more detailed explanation of the fraud."
Jennifer Calvery
Group Head of Financial Crime Risk and Compliance, HSBC
Read the full customer story here
AI-driven AML in practice at HSBC
HSBC is one example of a global bank leading the way in using next-generation technologies such as AI to improve financial crime compliance. Back in 2017, HSBC deployed Quantexa’s Decision Intelligence Platform to improve its AML programs across complex areas of the bank.
Quantexa’s Decision Intelligence Platform has helped HSBC to:
Build a strong data foundation
“Knowing who is transacting with whom is critical for HSBC investigators who want to view entire counterparty networks as they search for illicit activities,” explains Jennifer Calvery, HSBC’s Group Head of Financial Crime Risk and Compliance. For HSBC, that means putting together a picture of how individual customers behave and operate using Quantexa’s Entity Resolution and Graph Analytics technology.
“Decision-makers didn’t always have a direct line of sight into their risk exposure to see all of the connections between their counterparties…and their counterparty’s customers, vendors, and suppliers,” explains Kai Yang, Global Data CIO at HSBC. Now Quantexa is HSBC’s enterprise-wide vendor of choice for Entity Resolution.
Gain a contextual view of global customer and counterparty risk.
In 2017, Quantexa created a Global Social Network Analytics (GSNA) platform for HSBC, so the bank could analyze data with “… a global analytics solution that identifies potential financial crime by contextually analyzing customer, transactional, and publicly available data to understand a customer’s global network…” This view allows the bank’s human investigators to better conduct informed analysis of potential criminal activity. The GSNA platform replaced several of the bank’s legacy data analysis tools and won HSBC the Celent Model Bank Award for Risk Management.
Simplify complex banking AML practices such as Trade Finance.
HSBC’s Global Trade and Receivables Finance (GTRF) business processes over 5.8 million trade transactions a year for signs of financial crime — vastly more transactions and connections than on banking’s retail side. In an industry first, in 2019, HSBC deployed Quantexa’s AI-enabled customer surveillance system that uses big data, advanced analytics, and automated Contextual Monitoring to detect and disrupt financial crime in international trade.
To automate screening processes for complex investigations.
When cases are reviewed by bank investigators, Quantexa supports investigations by highlighting resolved entities and networks – reducing false positives, and shortening the time needed to close cases.
“The vast majority of what’s going on is completely legal and legitimate, so we’re looking for a very small sub-segment in this big group, and so we need that contextual picture,” says Calvery. “All of these factors are causing banks to take a second look at how they use AI to automate the screening process and flag suspicious activities that may be lurking in the dark corners of this highly complex transactional maze.”
The results of HSBC’s reliance on Quantexa contextual AML solutions are clear: The bank has continued to reduce false positives year-over-year and is finding new risks not detected previously.
“If I wanted to boil it down into really simple terms, we find more of those financial criminals who are exploiting our products and services and we find them faster, through tools like Quantexa.”
Jennifer Calvery
Group Head of Financial Crime Risk and Compliance at HSBC
See how HSBC is using Quantexa’s Decision Intelligence Platform to fight financial crime here.
HSBC also uses Google AML to provide the transaction alerting solution in its retail and commercial operations, helping the bank help clients in the financial sector meet compliance regulations for screening and reporting potentially suspicious money laundering activity. This is a great example of how a bank can use multiple complementary solutions for different areas of the bank.
The vision: 1+1=3
The collaborative capabilities of Quantexa are not locked to a specific provider of AI anti-money laundering solutions. Quantexa can work directly with banks that are building their own AML models. The future promises multiple pathways for banks looking to work with AI – from working with best-in-breed technology to building models in-house and integrating with technology from companies such as Quantexa.
For more information on how your bank can benefit from Quantexa’s AML solutions, get in touch.
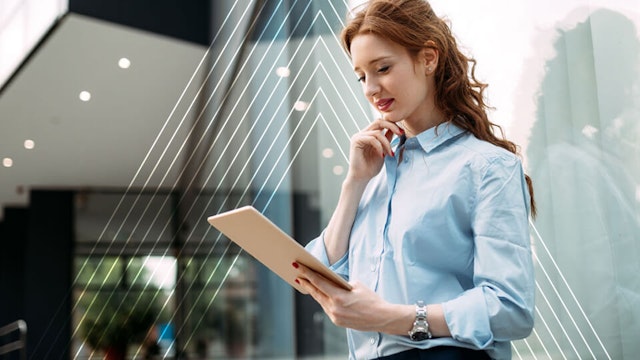