Embracing transparency, explainability, and interpretability for Responsible government AI in the U.S.
Ensuring AI technologies are trustworthy, fair, and beneficial for everyone while minimizing risks and maintaining public trust.
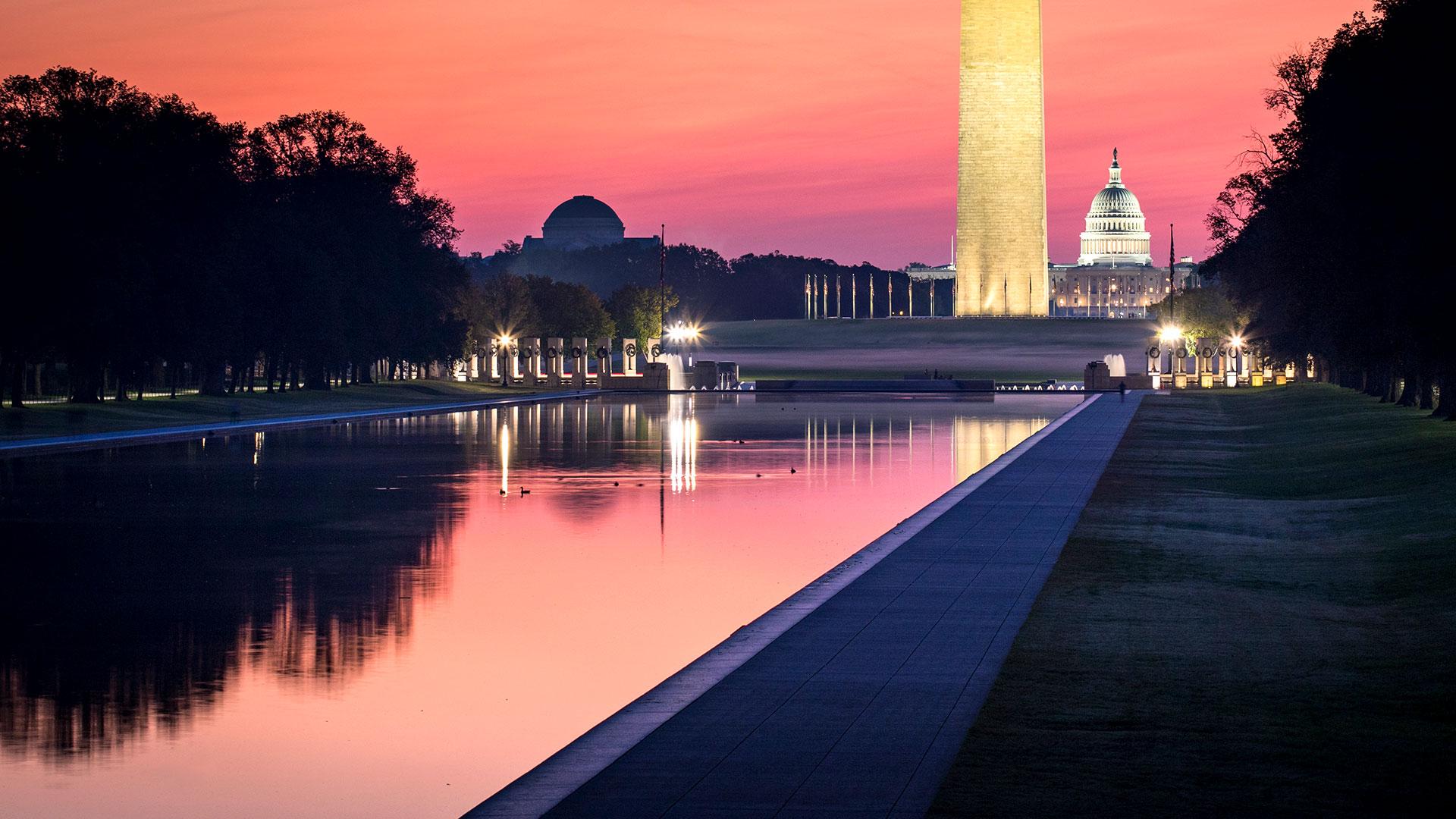
On July 26, 2024, the U.S. National Institute of Standards and Technology (NIST) released NIST-AI-600-1, Artificial Intelligence Risk Management Framework: Generative Artificial Intelligence Profile. This framework provides the U.S. federal government with a practical guide to design, develop, deploy, use, and govern AI in a manner consistent with respect for international human rights –what is called Responsible AI.
Transparency, explainability, and interpretability are distinct characteristics of AI systems that are often discussed in the context of responsible AI design and deployment. Let's look into what each of these terms means and why it's crucial for government AI buyers and designers to understand the differences to ensure their AI systems are the best they can be and are in compliance with federal guidelines.
1. Transparency
Transparency refers to the openness and visibility of the inner workings, processes, and data used in an AI system. It involves providing a clear picture of how the system operates at every level. Characteristics of a truly transparent AI system include:
Focus: Centers on the system's design, algorithms, and data.
Goal: Ensures stakeholders understand what the system is doing and why.
Example: Making code, data sources, or decision-making processes openly available for audit or inspection.
Transparency is critical for building trust, regulatory compliance, and ethical decision-making, as it enables external stakeholders (like regulators or users) to see the foundation of an AI system. However, transparency doesn't necessarily mean that the outputs are easily understood by non-experts.
2. Explainability
Explainability describes the ability of an AI system to articulate or provide reasons for its decisions in human-understandable terms. Explainability is especially important for high-stakes applications, such as healthcare or criminal justice, where decision-makers need clear and actionable insights to trust and validate AI recommendations.
3. Interpretability
Interpretability refers to the extent to which a human can understand the cause-effect relationship within an AI system. This is critical for systems that need to be validated or debugged by domain experts, making it easier understanding and troubleshooting without requiring significant external resources or tools.
Why these distinctions matter
Transparency ensures oversight and accountability but doesn't guarantee that stakeholders can understand specific outputs.
Explainability provides tailored insights into how decisions are made but doesn't inherently reveal system-level details.
Interpretability is about simplifying the model itself to make it intuitively understandable, which may limit the complexity or accuracy of the system.
Together, these characteristics address different facets of responsible AI deployment, catering to the needs of developers, users, and regulators.
Transparency, explainability, and interpretability with Quantexa
Quantexa addresses the distinct AI characteristics of transparency, explainability, and interpretability through our Decision Intelligence Platform, which emphasizes creating trust in AI-driven decisions. Here's how Quantexa approaches these needs:
Transparency: Quantexa integrates entity resolution and knowledge graphs to unify and visualize data. This process creates a clear representation of how decisions are derived from data, ensuring that users can understand the relationships and sources contributing to outcomes. Transparency is particularly crucial for regulated industries, as it ensures compliance and accountability in decision-making processes.
Explainability: The platform employs context-based learning, which combines diverse internal and external data sources to establish a comprehensive view of entities and their relationships. By presenting the rationale behind predictions and recommendations, Quantexa enables stakeholders to grasp the "why" behind decisions. This feature is critical for building trust and allowing users to validate or challenge AI outputs when necessary.
Interpretability: Quantexa's Composite AI approach leverages multiple AI techniques and domain expertise to avoid the "black-box" nature often associated with single-model systems. By breaking down complex analyses into understandable steps, the system ensures that users, regardless of technical expertise, can interpret and act on the results effectively.
These features allow Quantexa to support use cases like fraud detection, financial crime monitoring, and policy decisions in both government and private sectors while maintaining the trust of its users and stakeholders. Our focus on explainability and contextual insights has been recognized as a key differentiator for Quantexa among other decision intelligence solutions. Quantexa embraces responsible AI adoption for fostering trust among citizens, and laying the groundwork for innovation that is ethical, secure, and aligned with public values.
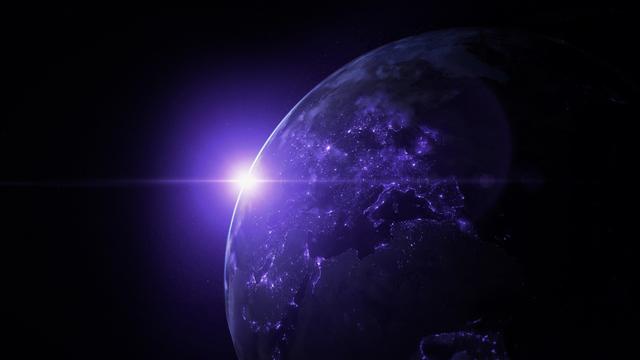