It's Not All Generative AI (But GenAI Helps)
Discover how generative AI and traditional techniques unite to elevate Quantexa’s composite AI for advanced decision intelligence.
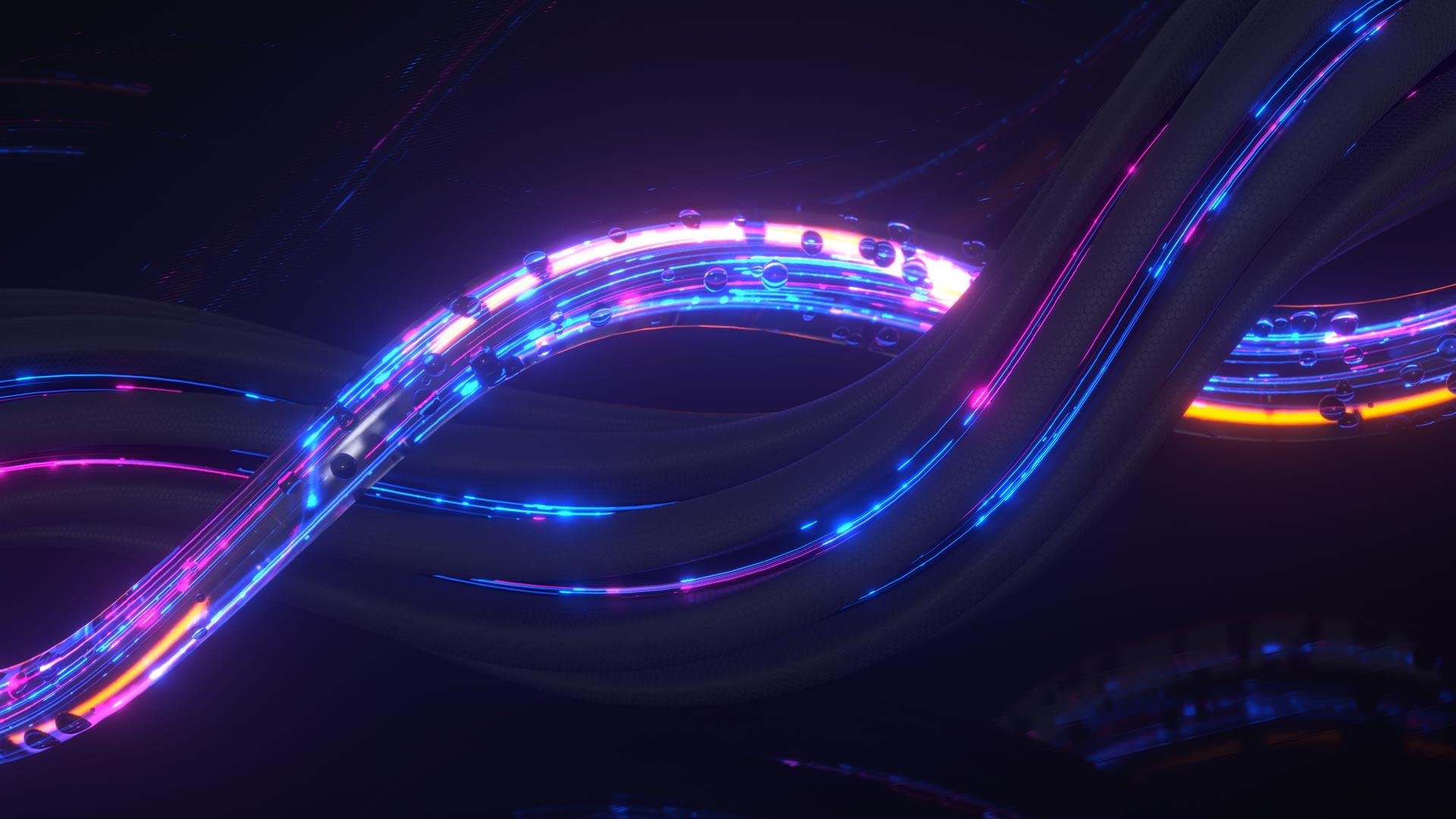
Generative AI is here to stay. It shows great promise, but in particular areas Generative AI is no silver bullet, and traditional methods continue to hold considerable value.
Gartner®recommends that “
The combination of GenAI models with other AI techniques can be particularly powerful.
The potential combinations of AI techniques are endless. Strong combinations and use cases include:
Nongenerative ML and GenAI models for segmentation and classification, synthetic data generation and computer vision
Optimization/search and GenAI models for enterprise search
Simulation and GenAI models for simulation acceleration
Graphs and GenAI models for knowledge management and retrieval-augmented generation
Rule-based systems and GenAI models for chatbots, robo-advisors and specialized natural language generation1.”
The importance of being….. composite AI
With Quantexa being a graph-centered technology, while GenAI has very much brought the knowledge graph to the fore, graphs and networks have partnered with AI for decades. For Quantexa, then, a composite approach to AI helps underpin its Platform’s model rigor, flexibility, and facilitate use-case tailored predictive workflows. Composite AI entails more than picking the right tool for the job, but also utilizing a complete toolbox for a range of jobs.
According to Gartner, “Composite AI refers to the combined application (or fusion) of different AI techniques to improve the efficiency of learning to broaden the level of knowledge representations.”2
AI is infused across Quantexa’s Decision Intelligence Platform, including
The fusion and natural language processing of structured and unstructured data sources to resolve entities, and building a contextual fabric driving models and decisioning.
Modelling and score-based decisioning, centered on expert knowledge and drawing on model sets to trigger and alert the Subject Matter Expert, e.g. fraud analyst or risk manager, to investigate a prospective risk.
Incorporating GenAI and LLMs to democratize analytics workflows for domain experts through copilot technologies, helping to consume and create content, for example Suspicious Activity Reports. Foundation models are also helpful for low parameter/low target problems.
In the following sections, to show the breadth of the Quantexa Composite AI capability, we show how supervised and unsupervised learning informs Quantexa detection model architectures, explore how graphs ground Generative AI Retrieval Augmented Generation (RAG) pipelines, and finally dive into a specific Shell Company detection implementation.
Supervised and unsupervised learning in Quantexa detection models
For detection, the Quantexa Platform assembles views to reveal detailed interactions, for example as transactional relationships or company affiliations. The derived context learnt directly from your data bedrocks the predictive AI methods powering the detection models. This in turn helps domain experts such as investigators by quickly taking projects from resolved entities and network intelligence directly to investigation.
Supervised Machine Learning methods, with sufficient historical data of known examples, empower the detection models to assess historical cases to help understand, review and automatically score large numbers of risk alerts. This can help augment scorecards with hitherto rarely observed but important risks.
For example:
Nearest Neighbours can underpin peer-based anomaly detection for churn detection in Customer Intelligence, and transaction monitoring for fraud detection.
Multi-hop risk detection helps identify high risk connected parties.
Transparent ML methods such as logistic regression and decision trees can drive and enhance scorecard tuning.
Unsupervised Machine Learning, meanwhile, can illuminate unknown patterns without recourse to known histories, for example, developing network and entity level features to flag and inform hitherto unidentified relevant predictive features, for example anomalous behavior in churn models and for transaction monitoring.
In financial crime, for example, supervised learning techniques such as gradient boosting (XG Boost), Support Vector Machines and Random Forests lend themselves well to high volume, long history of use cases, while Regression and Decision trees, alongside Hierarchical and K-means clustering (unsupervised machine learning) have traditionally serviced lower volume workflows, including those with fewer known historical cases. However, foundation models are proving useful to understanding the relationships between low parameterization and few targets.
In sum, it's not about the “best algorithm”, e.g. regression versus neural nets, since much depends on the data volumes and historical context available, while the presence of human knowledge - expert scorecards - can help provide great context. Models too don't sit standalone. For many decision-based processes, they augment. By extracting better model features from entity and network context, they can deliver a step change to results efficacy - reducing false positives and simplifying investigations. In one retail AML case, timing of historic investigations into targeted entities and counterparties highlighted useful temporal features.
AI thrives on the use case, data types and volumes, and knowledge of context. Quantexa, for its part, draws on its market expertise as well as the very best of data science to inform the detection methods in its Platform. This helps users, whatever their level of AI or domain skill, to adopt methodologies appropriate to their need.
Graphs and GenAI
We at Quantexa see GenerativeAI as an extension of traditional AI, part of the Composite AI. In addition, there is a natural alignment between graphs and GenAI, for example in Retrieval Augmented Generation workflows.
In this way, knowledge graphs pass grounded knowledge – organizational truth – through contextualized prompts to the LLM. As we have discussed elsewhere, knowledge graphs can help reduce hallucinations, augment, update and stimulate the pre-trained global knowledge contained in the LLM.
Composite AI in action: The Quantexa Shell Detection model
One particularly pertinent use case, particularly for Government agencies, is Shell Company detection, delivering 97% accuracy for example in the Singapore jurisdiction. It has been successfully deployed in both Financial Services and Government to identify 1000s of productive cases.
A Shell Company fraud is an umbrella term to describe a range of risky company behaviors. This includes Mini Umbrella Companies (MUCs), shelf companies, phoenix companies, and property tax. Illegitimate shells can be used for money laundering, tax evasion, or hiding the identities of their owners.
Shell companies share characteristics that can assist investigators, such as:
A short existence
A low number of temporary workers employed
Little or no financial information
The Quantexa Shell Detection tool assigns each company a probability score to indicate its likelihood of being a Shell. The Shell Detection tool is trained on Corporate Registry data processed by Quantexa’s Entity Resolution.
Its methodology uses a Semi-supervised model. The machine learning based tool is trained and tested on multiple jurisdictions (inc. UK and Singapore). Here, different models have been trained on different data sources, from Bureau van Dijk and D&B Open Corporates, with labels for each data source selected with assistance from Subject Matter Experts (SMEs)
The UK jurisdiction, for example, includes a wide variety of different types of shell companies. Because of this, even a limited number of financial features can dramatically affect model performance. Therefore, for the UK, the Shell Detection tool offers two separate models to train and score the financial Documents and non-financial Documents.
The algorithm used for every model is XGBoost.
The model is explainable – the key drivers for each prediction can be surfaced from the model.
Enhancing predictive intelligence
At Quantexa, Composite AI informs predictive models that drives decision intelligence. We have exemplified Shell company detection utilizing a semi-supervised set of models, and looked at how supervised and unsupervised models could automate and identify general detection trends that the human eye can miss.
However, we also see how prompt methodologies and the generative knowledge enabled through LLMs can inspire new ideas and summarize knowledge, for example for Suspicious Activity Reports, when grounded on organizational knowledge such as that provided by a knowledge graph. In this way, generative AI can facilitate predictive workflows when combined with predictive models and contextual intelligence.
1Gartner Article, When Not to Use Generative AI, By Ava McCartney, April 23, 2024.
2Gartner Glossary, Composite AI, 2024
GARTNER is a registered trademark and service mark of Gartner, Inc. and/or its affiliates in the U.S. and internationally and is used herein with permission. All rights reserved.
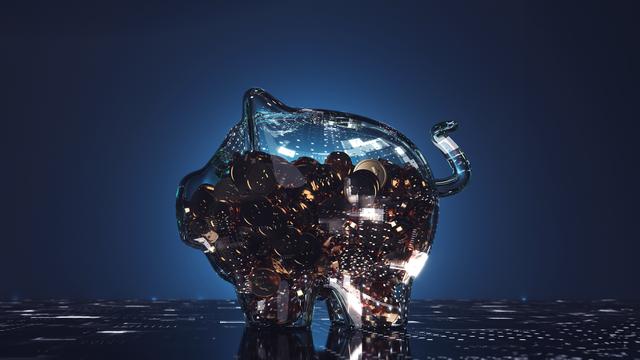