Knowledge Graphs and LLMs: A Practical Guide to the Next Frontier for B2B Sales
Large language models (LLMs) can unlock huge value for B2B sales teams, but for the best results, they require grounding in real-world, contextual data.
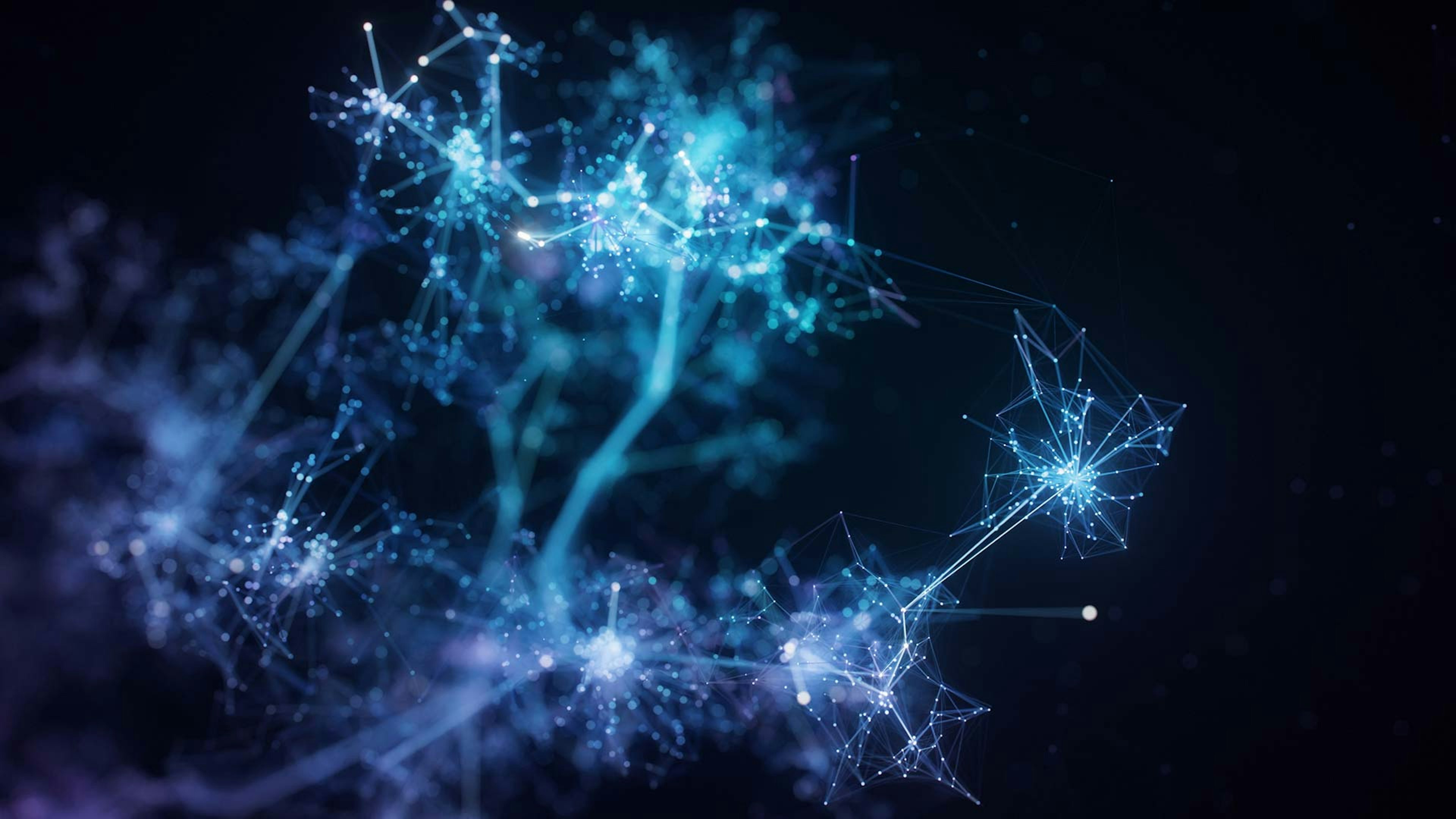
Sales and marketing is one of the areas where generative AI and large learning models (LLMs) have the potential to drive the most value. McKinsey estimates nearly $1 trillion of annual impact across these functions alone.
Similarly, a survey by Bain & Co. of B2B business leaders highlighted sales, marketing, and customer support as the areas where foundational models and generative AI have the highest potential. BCG research found that 70% of sales leaders plan to invest in Generative AI.
So, there is broad consensus on the potential impact across these functions. But what are the practical implications of implementing these technologies in B2B sales? How can organizations combine state-of-the-art technologies like LLMs and knowledge graphs to unlock even greater benefits? And what are some of the challenges organizations face in doing so?
These are some of the questions that we'll unpack in this blog post.
What's the opportunity for B2B sales?
B2B sales teams have a tough job. They're typically dealing with complex clients in highly competitive sales environments, and need to have a deep understanding of a wide range of products and services. With so much on their plates, it's critical to know which clients and prospects to talk to, about what, and when.
Data has emerged as a key differentiator over the last decade or so to drive efficiency and growth in B2B sales. For example, to identify high-value new customer acquisition opportunities, or to proactively engage clients about certain products or services. But data-driven solutions have typically lacked either flexibility or speed—for example, generating real-time alerts and leads based on static, predefined models don't help sales teams react quickly to changes in the market, and ad-hoc requests to analytics teams can take days, weeks or even months, by which time the opportunity has often passed.
New technologies such as LLMs provide the opportunity for a paradigm shift, where sales teams have all the data and information they need at their fingertips to drive proactive and meaningful engagement.
For example, a commercial banking relationship manager could simply ask some questions—"Who are the most important suppliers in the car manufacturing industry in China? Which of these are already clients of the bank?" or "Which of my clients are most likely to be interested in our new foreign exchange app? Generate a personalized email for each client"—and immediately receive a response.
Or a Telco account manager could ask, "Which of my clients have been impacted by the recent network outage in South London? Whom should I contact first?" or "How much is client ABC spending on network infrastructure? How does this compare to other, similar companies?"
What might have seemed like science fiction just five years ago is now possible using the latest technology.
What are LLMs and knowledge graphs? And why combine them?
Large language models are machine learning models that are capable of understanding and generating human language and are pre-trained on vast amounts of data. ChatGPT is an example of a commonly used service powered by OpenAI's GPT LLM.
Knowledge graphs are a way of storing data that represents real-world entities, and the relationships between those entities. Knowledge graphs can be used to represent complex real-world systems and events like ownership structures, supply chains, and network infrastructure.
On their own, LLMs are great at answering generic questions using the vast amounts of publicly available information they've been trained on. However, more specific knowledge is required to provide real value in a commercial context—including your internal data, which LLMs lack. For example, an LLM would not be able to answer questions relating to a particular customer's product holdings or interactions, as it does not know this information.
Knowledge graphs can contain huge amounts of useful, domain-specific information and provide a very strong basis to unlock contextual insights by data science teams. They can be difficult to interpret at scale by business users because of the number of nodes and edges, and the complexity of relationships. For example, a knowledge graph in commercial banking might represent a bank's global client base and their supply chains, enriched with firmographic details and news events.
Knowledge graphs also require a foundation of good quality, connected data to provide an accurate reflection of real-world relationships, which can be created through entity resolution.
Combining LLMs with knowledge graphs can provide you with the best of both worlds—a simple, natural language interface powered by a broad set of contextual, domain, and organization-specific data.
The grounding of LLMs in the knowledge graph data not only reduces the likelihood of hallucinations but allows traceability of outputs to real data points contained within the knowledge graph. This is crucial in building trust with users and auditability for commercial applications.
Knowledge graphs can also contain recent and even real-time data, whereas many LLMs have been trained only on data up to a certain point. (For example, GPT-4 Turbo has a cutoff date of April 2023.) This is essential for B2B sales, where you need up-to-date information on the organization and the people you're dealing with—for example, customer info, product holdings, news, or recent interactions.
What's the business value?
There are 4 key areas of value that enabling B2B sales with an LLM and knowledge graph–powered assistant can unlock:
Revenue growth:
Accelerate lead generation. Access to richer information via a conversational interface enables sales teams to identify more leads at a faster rate
Improved conversion rates. Sales teams are equipped with the right information and prompts to drive personalized and proactive engagement and help close deals
Efficiency:
Increased sales team productivity. Sales teams no longer need to spend time synthesizing disparate information or requesting data from other teams
Increased analytics team productivity. Analytics teams save time dealing with ad-hoc requests and can benefit from a knowledge graph foundation for modeling
Client and employee experience:
Enhanced client experience. Clients receive timely, relevant engagement, with no unnecessary fact-finding from sales teams
Enhanced employee experience. Sales teams get to focus on sales strategy and client engagement and are upskilled in the latest tech
Reduced risk:
Improved risk identification. Sales teams can better understand associated risks as well as opportunities—for example, credit shocks in supply chains, or links to sanctioned entities
What are some of the practical challenges?
Responsible AI and governance
Having the right guardrails in place around any application of generative AI is critical. In B2B sales, there is usually a member of the sales team interpreting the outputs rather than these being surfaced directly to a customer (such as via a B2C chatbot), so the risks of hallucinations or inappropriate information being shared are somewhat reduced. However, it is still critical to ensure that:
robust testing is conducted;
effective moderation, monitoring, and feedback loops are in place;
safety, privacy, and access controls are embedded;
users are aware of model limitations.
Microsoft and Google both provide useful capabilities here, for example, Azure AI Content Safety and Gemini API Safety Attributes.
Trust, adoption, and upskilling
To ensure the potential value of these technologies is realized, sales teams need to know how to use them effectively and be willing to do so. The key here is ensuring that these systems are designed with the sales teams in a user-centered way, so they are involved in the development process and the solution is one that they can quickly get value from. Providing training on how to use the system is critical, but so is training on how generative AI works, and the limitations users must be aware of.
Public vs private LLMs
One issue many organizations have been grappling with is the use of public vs private LLMs, given the limitations on sharing confidential company data with public models versus the cost and effectiveness of training and hosting private LLMs. The rise of Retrieval Augmented Generation (RAG) and more mature and secure services becoming available from the likes of Microsoft and Google are now enabling more companies to combine their private data with public LLMs.
Continuous innovation
Given this is such a rapidly evolving space, it is important that companies retain a level of flexibility in their approach where possible, to enable them to rapidly adopt and benefit from new versions of LLMs as they are released in the future. This flexibility will require continuous and close collaboration between technology, data, and business teams to identify, implement, and adopt the latest technologies efficiently and effectively.
Summary
The potential of LLMs and knowledge graphs to transform B2B sales is clear, and the benefits will be significant. There will be some challenges in implementations, particularly with regard to data privacy, governance, and adoption, but one thing is clear: Those who don't act now risk being left behind.
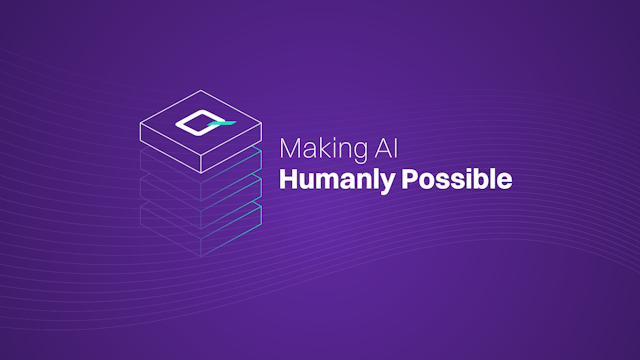