Reimagine Detection and Monitoring to Optimize Alerts
Instead of trying to fix an inefficient process, is it time to reconsider how monitoring and detection are fundamentally performed?
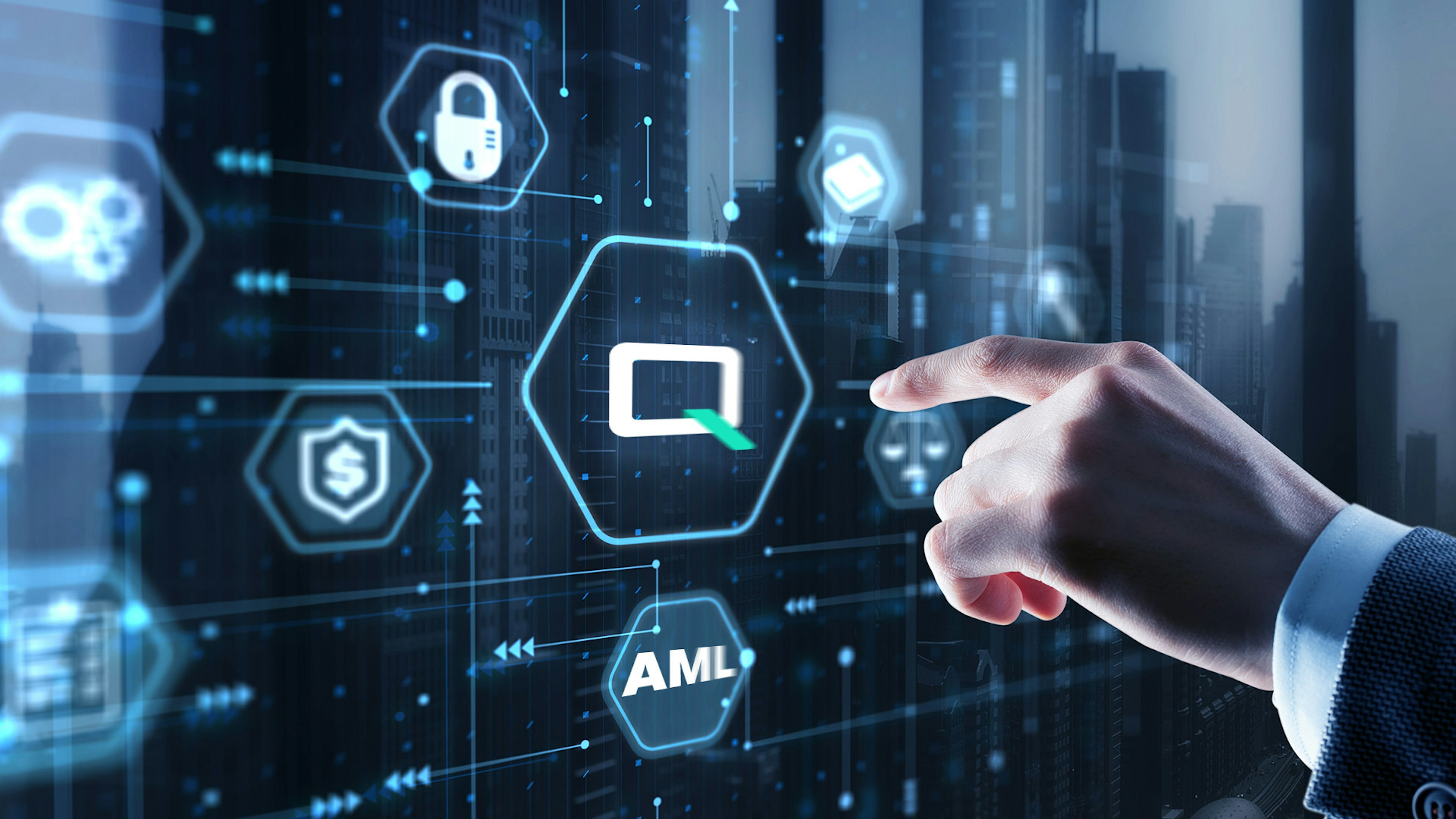
People are always keen to explore fresh perspectives in the dynamic field of AML transaction monitoring. Rather than focus on false positives, this article delves into a thought-provoking concept that aims to inspire innovation.
Since the first transaction monitoring solutions were introduced more than 20 years ago, relatively little has changed in the way those solutions work. At the time they were developed, the systems represented the maximum level of functionality and automation achievable by the existing technology for the given detection processes. The basic premise was to load transactions into a database and then run a series of rules against a given time period, looking for activity that exceeded predetermined thresholds in order to generate an alert.
Over time these rules were combined with others, and eventually some optimization was layered on top of them in order to focus on alerts deemed higher priority. And while some rules, like those designed to identify structuring, are effective, others were much less so. Sound familiar? Does your current solution fundamentally still work in this way?
Let's take a step back. What were these rules initially designed to do? When these first rules were created, they were meant to look for indicators of the proceeds from criminal activity as they were being laundered through the financial system. Think of the FATF recommendations: a noble and worthy endeavor. But as you well know, the one-size-fits-all approach to these rules was fraught with challenges.
What can be done differently?
What if there was a paradigm shift in monitoring and detection to align more closely with identifying activity related to specific predicate criminal offenses? After all, that was the initial intent in creating those historic rules. But instead of running a series of rules, hoping to identify transactions that exceed a threshold and trying to understand what they might mean, you could create more granular approach.
What if we broke down the activity into smaller parts which, when combined, would indicate a specific typology tied to a known predicate criminal offense? These typologies are defined as specific methods or schemes that are used for laundering money and other financial crimes — trafficking of humans or drugs, mule activity, hawalas, and many more. Each typology has different red flags and indicators, based off regulatory guidance and historical outcomes that generate precise alerts linked to those specific crimes. This would eliminate the time needed to try and understand what those historically generated alerts were indicating.
As a byproduct of this more granular approach to monitoring, different attributes could be utilized to distinguish a victim versus a perpetrator within the transactions. Doing so would help the banks better assist those in need and close the accounts of the bad actors.
Advances in technology make typology-based detection a reality
Data loads and processing times have become quicker and cheaper. Leveraging entity resolution and network analytics in the monitoring process provides a consolidated view of entities while exposing relationships that might not otherwise be apparent. Connecting parties through relationships and transactional data brings clarity in identifying where transactions originate and where the funds are sent to, making it easier to follow the flow of money.
Once the network view is generated, layering typology-driven detections over the network produces optimized alerts. In this way, the alerts will provide insights into the conductors, the volume of activity, and a direct link to the suspected crime, which no other detection method does today. If done correctly, the results could mean a vast reduction in time and effort spent triaging alerts and shifting the focus to the investigation process.
By harnessing technology to look at monitoring and detection from a new point of view — through predicate typologies — humans can do their jobs more effectively and efficiently. This technological and human partnership provides value by pinpointing specific risks and uncovering the unknowns through automation, which in turn allows the humans to focus and spend more time on complex investigations tied to criminal behavior — the work they were hired for in the first place.
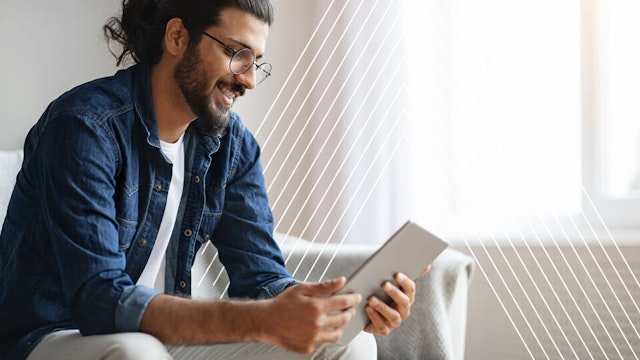