Unlock Insights From Adverse Media Using Contextual Search for Unstructured Data
Unstructured data can be costly and time-consuming to search. But using contextual search tools makes the job more manageable and cost-effective.
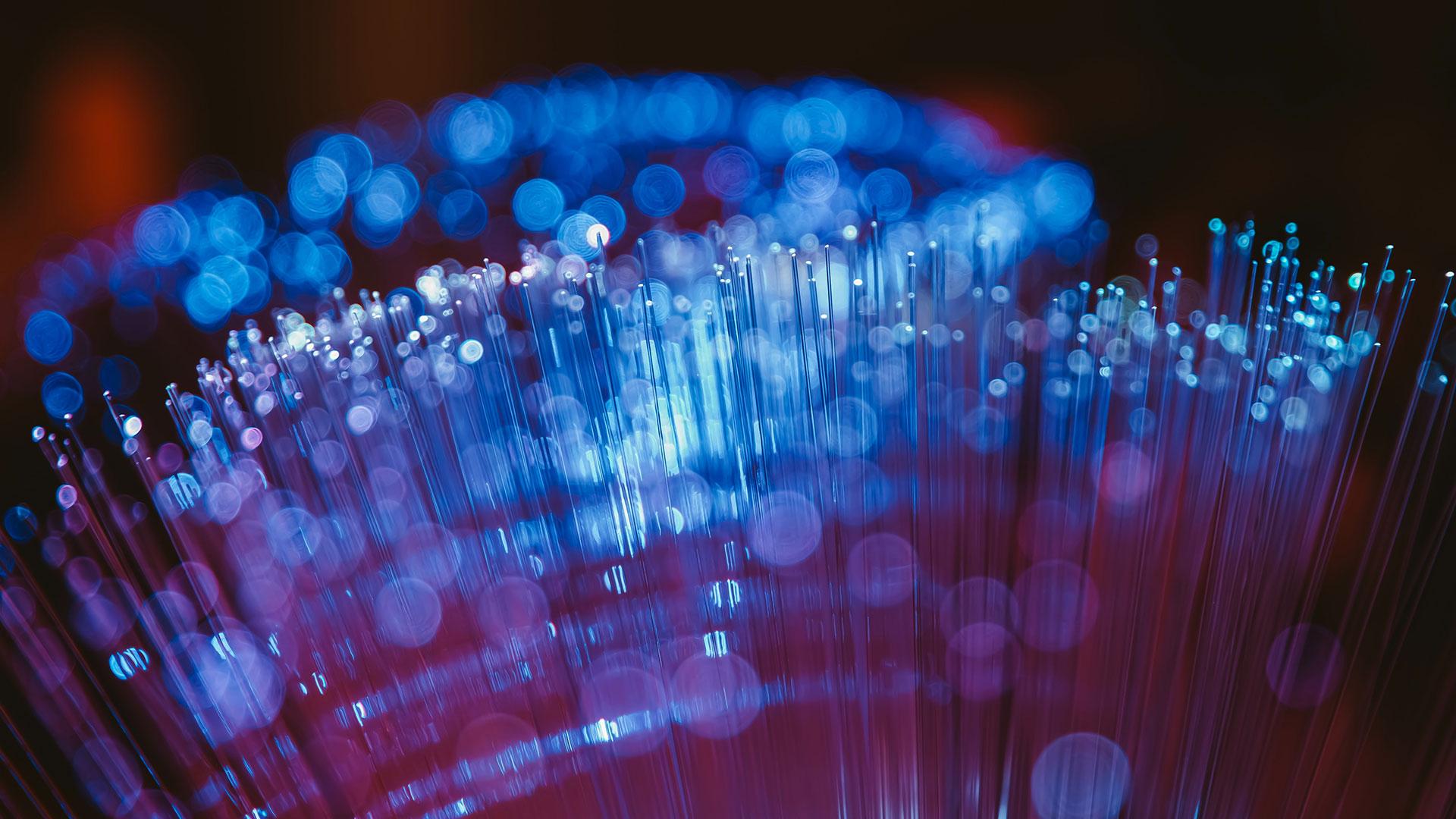
Today, unstructured data accounts for up to 90% of all generated data and is growing at an average rate of 60% each year. This makes it more important than ever for organizations to find innovative ways of managing and analyzing that data so they can utilize it effectively to enrich investigations and make trusted business decisions.
However, unlike structured data – which can be displayed in rows, columns, and relational databases, and is easier to manage and protect in legacy systems – unstructured data is much harder to use and extract intelligence from. But the deep insights that can be derived from unstructured data makes it a gold mine for forward-thinking organizations.
What is unstructured data?
Any type of information that does not have a recognizable, pre-defined data model or is not organized in a pre-defined manner is considered unstructured. Some of the most common examples of unstructured data include:
News stories & articles: Data from news stories and articles tend to be very text-heavy and descriptive, containing a wealth of rich information about people, businesses, and the activities they are associated with.
Intelligence case files: These reports typically contain lengthy text descriptions about people, businesses, and the activities they are associated with, and like news stories, can hold a ton of value.
Emails: While emails include some structured data (for example, the sender and recipient email addresses, dates and times, and subject information), the body text fails to follow a recognizable format.
Challenges in attempting to contextualize unstructured data
When attempting to contextualize unstructured data, there are two approaches that can be taken. One involves using Natural Language Processing (NLP) to perform entity extraction accurately across all data sources. The other involves taking a set of known "entities" and manually searching for them within the unstructured data, which is very labor-intensive. Plus, extracting entities from unstructured data is only half of the challenge.
Organizations need to be able to make full use of the entities mentioned within the unstructured data by linking them to the existing corpus of data. So, the only way to truly make sense of unstructured data – and leverage it to make better operational decisions – is through automation, and by applying context.
Applying context to unstructured data
Unstructured data has value across multiple areas, from finding bad actors and understanding credit risk decisions to improving customer service.
For instance, many organizations in industries like banking, insurance, and government, are now required to incorporate unstructured sources such as adverse media (also known as negative news), unstructured case files, and intelligence reports to make meaningful business decisions. A number of their key operations rely on this data: anti-money laundering, fighting financial crime, fraud investigations, risk decisions, and customer onboarding.
But they face a challenge: Due to the unstructured nature of this data and their lack of clear identifiers, it’s difficult to automatically map this to an individual or a business of interest.
This results in inefficient and inaccurate manual processes, such as relying on online search engines, or on tools that in turn rely on simple name matching. This can lead to a high number of false positives, resulting in operational inefficiencies, poor customer experience, regulatory sanctions, and missed revenue.
But harnessing the power of Entity Resolution and network generation capabilities presents a solution to these challenges. This is known as contextual search for unstructured data.
What is contextual search?
Contextual search is a new product within the Quantexa Decision Intelligence Platform that focuses on the context of the query, as well as on the intent of the user, to fetch the most relevant set of results. It enables investigators to identify more accurate matches within unstructured data at speed, thanks to the single entity view of an organization’s internal data combined with the relationships that exist within it. This provides more context for the matching process and leads to more accurate results.
By utilizing the bulk of an organization’s data that is often left unused, the efficiency and effectiveness of your processes can be improved, and you’ll benefit from much greater levels of accuracy.
Contextual search in action: Who is "Jamie Hutton"?
So, how does Contextual Search work? Here, we outline the process:
You are searching for information on a particular customer – "Jamie Hutton" – and you have extensive data on them, including their date of birth and national insurance number.
You might trawl through news articles, online blog posts, or adverse news databases. But the best you will find is a name and age match, as the article or blog post is unlikely to contain any other identifiers.
This creates a problem. No amount of AI or machine learning will tell you which search results are relevant to the customer in question.
The only way to get accurate search results is by adding context. You need to understand who "Jamie Hutton" is in relation to the other data that is available. You might find publicly available sources, like corporate registries where you would learn that "Jamie Hutton" is a director at a company called "Quantexa," which is registered in London, UK, and that there are other directors, including "Vishal Marria," "Imam Hoque," and "Alexon Bell."
Matching data in this way makes a fundamental difference. Now, you are not solely focusing on the details pertaining to "Jamie Hutton" himself, but also on the details of the context around "Jamie Hutton." This enables you to derive highly accurate results and enriches your investigations with relevant information.
Why contextual search is the future
Contextual search helps organizations find the most relevant information from a vast amount of unstructured data — meaning it helps organizations derive the most value from their data.
In today’s highly competitive business landscape, the organizations that will prosper are those that make use of all the data that is available to them. Tapping into unstructured data is central to this, and contextual search is making it possible.
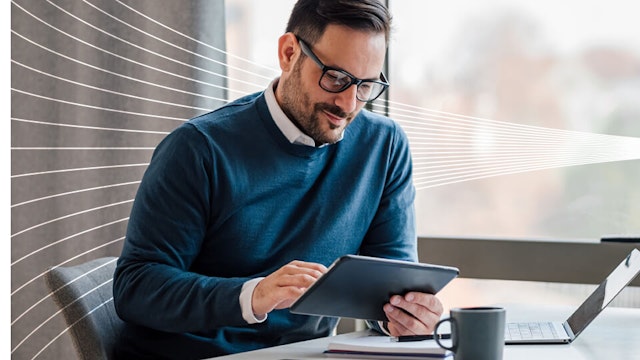