It’s Time To Upgrade Your Early Warning Signal Systems – Here’s How
Accelerate your early warning signals and mitigate risk using Decision Intelligence
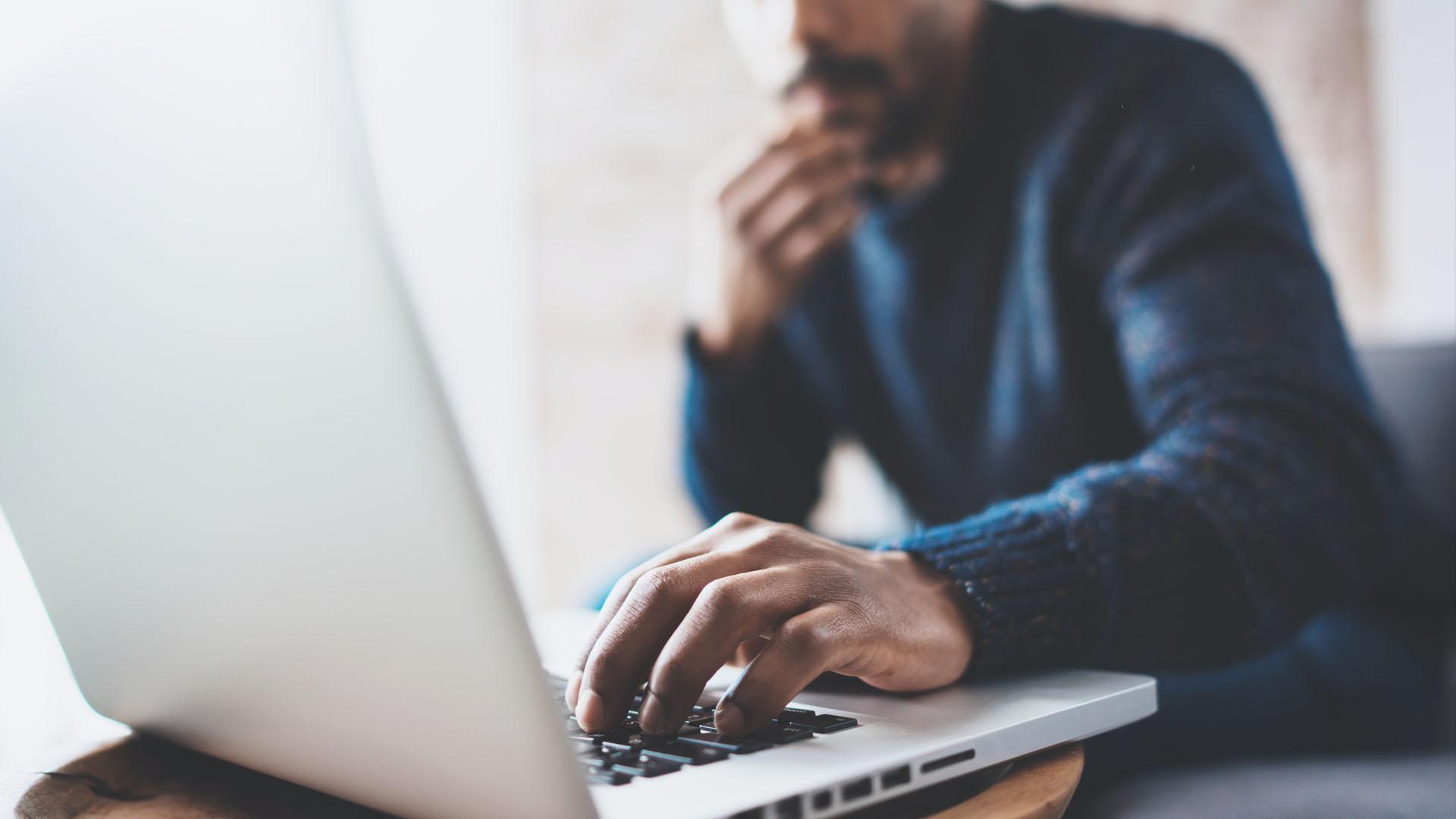
In recent months, the financial press, industry bodies, and analysts have warned of the risks of a looming recession. While no one can predict exactly when that will happen or how long and deep the recession might be, the consensus is that a global recession is likely to happen sometime in 2023.
For those lending to businesses, adjusting risk tolerance as financial markets change is critical. In today’s environment banks who had the foresight to invest in superior early-warning tools now have an advantage over those applying more traditional approaches.
With the possibility of a recession on the horizon, the risk cycle is changing and as Moody’s CEO, Rob Fauber, recently discussed with Quantexa’s CEO, Vishal Marria, the reasons are multiple, complex, and interlinked.
Companies need to upgrade legacy Early Warning Signal (EWS) systems to better predict when changes in the marketplace may occur. More importantly, however, they need to see individual customers and enterprises in context by being able to visualize all of the connections between their customers and who they are doing business with to make better lending decisions.
The problem for many financial institutions, however, is that data across the organizations sits within siloes. In addition, most institutions lack the technology necessary to bring these siloed datasets together to provide a holistic view of companies and individuals to ultimately understand:
Who are they doing business with?
What risks do they need to know about an enterprise with which they are going to do business with? i.e. physical risks as part of climate risk assessment
Is there appetite to continue doing business with that enterprise? i.e., is the risk well understood and acceptable?
Are there risk concerns within any part of a complex group? i.e., are trade finance performance issues emerging?
“Do they want to do business with that organization.” i.e., are there reputational risks that may be inherent in things like adverse media?
Credit cycle is turning, but this time it’s different
Global economies are under pressure having suffered four linked shocks since 2020:
The Covid-19 pandemic
A huge fiscal and monetary expansion
Pent-up demand post pandemic hitting supply constraints in industrial inputs and commodities
Russia’s invasion of Ukraine, which has driven energy prices higher.
This has resulted in more uncertainty and high inflationary pressure in the US and more pronounced inflation in Europe. Central banks are tackling this with aggressive monetary tightening, which may contribute to the possibility of a recession next year. This is already having an impact and in England and Wales, by June 2022, corporate insolvencies had hit a 13-year high.
Certain sectors are more impacted by the high input costs, energy, and commodities, including construction, retail, food producers, and manufacturing. Many companies are also at the mercy of supply chain disruptions, as noted by the Bank of England’s recent analysis. This report identified supply chain bottlenecks being driven by a range of issues including covid-related lockdowns in some key producer economies.
Higher funding costs and the indirect impact of global inflation surges, compounds challenges for corporations. Finally, climate-related risks, particularly physical risks, are hitting portfolios earlier than expected. This was seen in Australia where insolvencies jumped by 27% and 34% respectively in NSW and Queensland, due to localized flooding.
It’s clear that to have the best view of emerging risk, financial decision-makers need to holistically assess a range of connected risks.
Current EWS tools and processes are not fit for purpose
Most relationship managers and risk analysts are not set up for success. Partly due to historic systems underinvestment, they must navigate a range of siloed internal databases and then manually enrich this with external data.
By looking only at single datasets, key risk information may be missed. Reactive monitoring of EWS results in an inconsistent and manual approach, plagued by false positives. EWS processes are fitted around other credit processes, which already have an estimated 50% of time spent on admin or data wrangling.
Risks don’t occur in the company itself, they happen to the company. EWS tools need to assess all stakeholders in the customer ecosystem, which can only be done by evaluating multiple datasets together.
Decision Intelligence—A paradigm shift for better EWS
To move the needle, financial decision-makers need:
A single view of customers and counterparties
A networked view of their ecosystem
The ability to score and alert multiple risk types
These three necessities unlock a wider and more realistic view of the customer and their risk drivers. They also allow risk assessment to extend deeper into the horizon to see incoming risks from farther away to better protect the customer portfolio. Here’s how it works.
Most enterprises have all the data they need to understand their customers and their risk. The problem is that data is generally inaccurate and poor quality. Single-view solutions can’t handle data lake volumes, and quickly become siloed to specific use cases.
Without good data, models underperform and can lack transparency, so businesses can’t confidently get the full view of their customers to decide whether they remain a sound lending risk or not.
Quantexa’s Decision Intelligence (DI) platform is a new generation of software designed to connect billions of data points across internal and external data sources, to provide a single view data hub enriched with vital intelligence about the network of relationships between people, organizations, and places.
How to create a single contextual view
Quantexa’s Platform allows decision-makers to join internal customer records with external data with a proven 99% accuracy. These techniques work at scale; some client deployments cover the entire Wholesale Division with records totaling more than 60 billion.
The platform utilizes contextual analysis to create a complete network graph of real-world entities and their links to internal and external data. It then finds relevant features and patterns experts can recognize and contribute to.
Entity Resolution links internal and external data to gain a complete picture of real-world entities – people, organizations, addresses, telephone numbers, internet devices – and their transactions and behavior.
Network generation automatically finds sets of resolved entities and links that are relevant, filtering out low-value ones and expanding others.
The Platform enables financial decision-makers to use context alongside raw data to boost features, rules, and models that identify and score opportunity and risk. Experts contribute to transparent contextual models by identifying patterns based on their experience.
Key benefits of Quantexa's Decision Intelligence Platform
The advantages of being able to visualize customers and businesses in a single view cannot be understated. Whether the client is a small SME with a simple term facility or a large multi-national holding syndicated revolving debt, it’s crucial to rapidly show a holistic view:
For RMs – benefits include pre-populating reports for a quicker client assessment. Within KYC, Quantexa pre-populated forms with 80% of required data, saving data inputting time.
For Credit Analysts – joining multiple internal systems to show a holistic view of exposure and collateral across multiple product lines offers a quicker view of the aggregated risk profile.
For Risk Modelers – having the relevant risk data already joined up, frees up time from data wrangling to risk analytics, using a broader set of enriched and linked data.
Enable earlier and more accurate risk discrimination
Quantexa’s EWS approach incorporates a wider range of data sources, including dynamic transaction data to build out a view of the supply chain and broader ecosystem. The existing bank-constructed financial and behavioral models are applied more widely from just the borrower customer to include key counterparties.
Taking this wider approach results in strong performance. In one project, Quantexa’s technology saw the window of early warning signals extended to over 12-18 months vs client tools. One significant benefit to applying non-correlated network features is that the accuracy rate is increased, reducing false positives. This adds meaningful lift to existing models; in one case Quantexa’s platform achieved double the Gini (accuracy).
Lower ROI and re-use the same platform for multiple use-cases
Quantexa provides customers with a single platform to serve joined-up internal and external data across the enterprise. Separately ingested data sources can be dynamically queried as graphs or entities. This opens a range of additional use-cases for multiple stakeholders, using the same data. For example:
1. Incorporating non-financial risk alerts into the Credit process
Quantexa’s technology has traditionally been applied to financial crime detection. For example, clients who already have trade finance data and scores to detect fraud or AML alerts, these triggers can be shared with the Credit Risk teams to assess the customer and counterparties for early signs of risk deterioration.
2. Fast scenario analysis
One client recently wanted to understand the corporate lending portfolio’s direct and indirect links to Russia, Ukraine, and Belarus. Using Quantexa, powering a partners’ dashboard, it was performed 900x faster. The alternative approach involved re-allocating 10 analysts from daily duties and spending 2 weeks to manually review less than 107% of the portfolio.
3. Re-focusing risk detection into opportunity identification
A key benefit of an optimized EWS tool is better segmenting of good vs bad customers. Banks can lend optimally to customers who initially look risky but operate in a strong and diversified ecosystem.
Doing nothing is not an option
The external environment is increasing in complexity, uncertainty, and risk. For banks lending to corporations, it’s important not to be last to identify and respond to a change in risk.
For overworked RMs and analysts, the last thing they want is another non-integrated EWS tool pinging large volumes of false positives. Fortunately, CDI is the powerful technology available to solve this now.
It provides confidence and trust to accurately create a single view across siloed internal and external data sets. Adding context distils insights into relevant risks with the combined network effect of reducing false positives.
Transaction data moves assessment to a proactive and dynamic approach, enabling client assessment at a holistic ecosystem level, to see incoming risks from farther away.
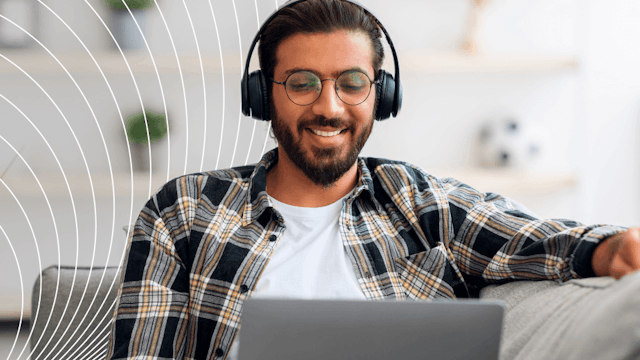