How to Operationalize Your Data for AI and Analytics
Building a trusted data foundation is the key to unlocking the full potential of AI, analytics, and smarter decision-making.
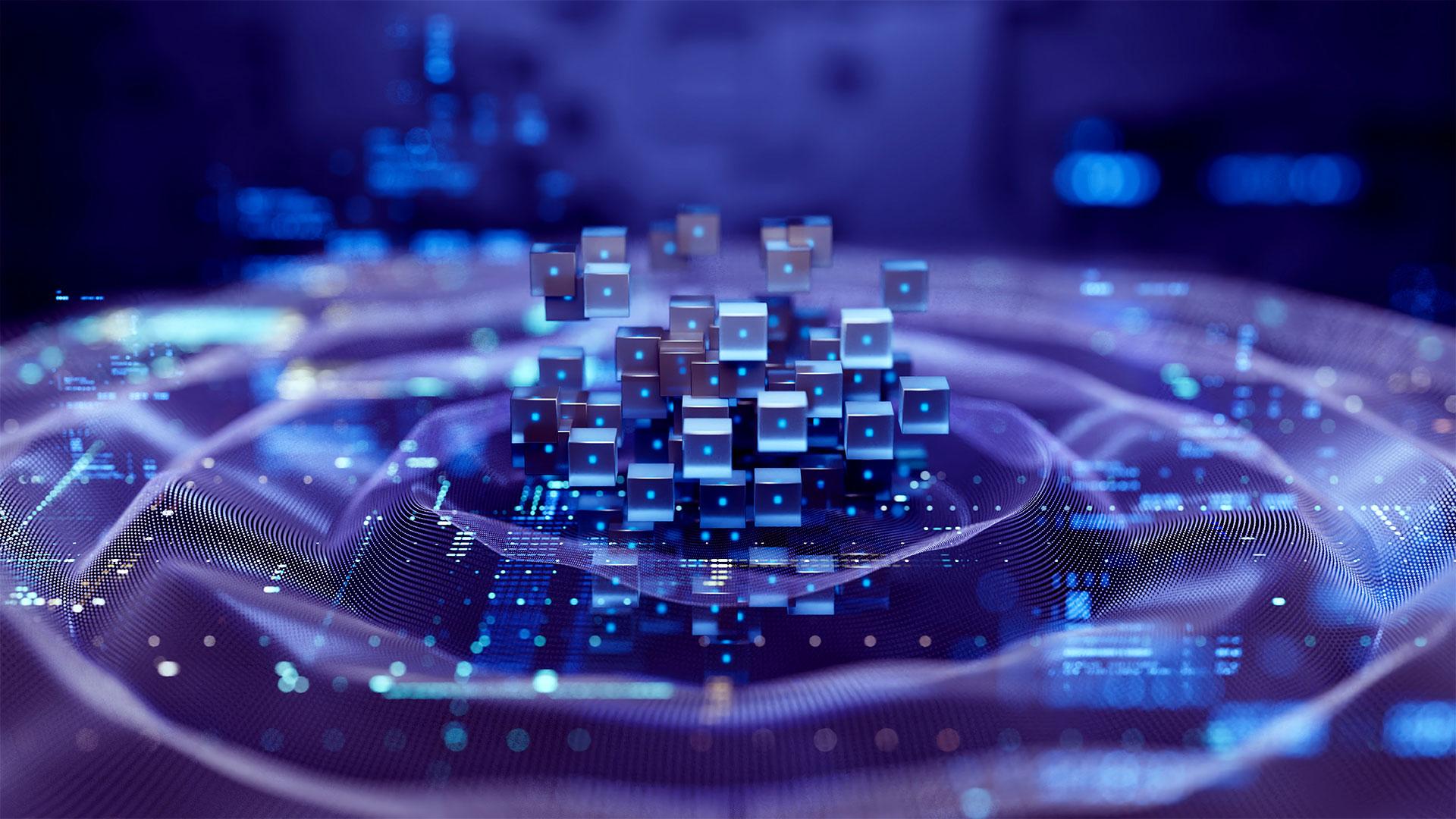
As your organization relies more on analytics for insights and decision-making based on artificial intelligence (AI), the need to bring your data together in a cohesive way becomes a business imperative. Data lakes are good for ad-hoc analytics, but don’t deploy neatly to end users. To get value from your data, it needs to be able to be put to work—that is, the right data must be accessible to those who need it, consistently, and in batch or in real-time. Only when these conditions are in place are you set up for AI and analytics success, and for true data-driven decision-making.
However, getting value from your data can be a significant challenge, particularly as organizations often find it’s ambiguous, siloed, or of poor quality. Moreover, true efficiency and return comes from leveraging your data across multiple use cases across the organization, and that presents a whole set of new challenges.
Particularly as organizations leverage greater and greater amounts of data to drive their business processes, operationalizing that data is crucially important—yet, some consistent obstacles stand in the way of companies being able to use their data effectively.
Challenges of operationalizing data
Clean, deconflicted, ready-to-use data—that is available to the right users across your organization, the moment they need it—is necessary to support real-time decision-making through internal channels, like your customer relationship management (CRM) system, or through external channels like online services.
But to take advantage of AI and analytics at this level, you need a strong data foundation that you can trust. Building that foundation is essential to getting past common roadblocks to using all your data effectively, like:
Data lakes as dumping grounds. Data lakes often contain a chaotic mix of raw data from multiple source systems, organizations, and third parties. Creating a joined-up view is a struggle, and because data processing is done in batch, they aren’t able to serve up real-time data for decision making. So, while data lakes can be useful for ad-hoc analytics, their data is not operational.
Poor data quality. Data accuracy and consistency issues tend to be chronic problems that arise when data is pooled from across multiple systems and over long spans of time. Poor data quality affects trust in data, which also undermines decision-making.
Complexity of data pipelines. Pulling data sources together to create a combined view requires a lot of complex work for just one use case. Eventually, organizations end up with siloed solutions for several key use cases. The challenge then becomes figuring out how to bring the data together while trying to serve up multiple use cases.
Limitations of operational data stores. Operational data stores often use relational database technologies—and increasingly, data stores such as Elasticsearch—to publish data assets for operational use. They provide a level of resilience for consuming applications – a helpful alternative to using data lake technologies, which are not operational. However, joining up data in the data pipeline and maintaining it remains a challenge.
Lack of scalability with data warehouses. Data can be joined together in data warehouses and used for reporting. However, these systems are often supported by platforms that don’t scale, which is a problem as the volume of data grows and data requirements evolve.
The need for an effective data management solution
Because of the many challenges commonly associated with creating a strong data foundation, companies must take stock of whether their data management solution is working for them—not only for use with AI, but for other advanced analytical projects, data-driven decision-making, and operational efficiency as a whole.
Considerations such as flexibility, scalability, and transparency are critical: Is the architecture sufficiently open that you can use data and models the way you need? Will the platform provide you with multiple views for different use cases? Is the platform open and transparent to end users and data teams? Will it scale with organizational ambitions, now and in the future?
Organizations must consider factors such as time to value: How long will the solution take to deploy? Will it require data to be transformed into a certain schema in order to be ingested? If so, could this process set you back significantly in time and cost, ultimately leading to project overrun?
And, of course, data matching accuracy must always be top of mind. Not only must the solution solve data quality problems, it must also join siloed data in order to create the trusted data foundation that can be fully operationalized for successful analytical endeavors.
Set a course for AI and analytics success
To make your data useful for AI and analytics, you need to join up data from multiple sources and make it consistently available for operational and analytical use.
One aspect that can be singled out that is critical for operationalizing data effectively would be the quality of data matching. For example, Quantexa’s Entity Resolution capability really stands out because it takes all the data you have available into account and iteratively works with it to find all the ways you can identify entities such as people, organizations and companies, giving you a trustworthy 360 view of your customers or other entities.
Because of the iterative matching process, you can make connections between data, even when data quality is low or where there has been manipulation.
Another thing to think about is how you are going to scale the matched data across different use cases where you typically need slightly different views of your data. In an AML scenario, where you are trying to uncover hidden transaction flows, your matching rules (fuzziness levels) will be much looser than, say, in Marketing where you are subject to strict GDPR regulations. If data matching is done dynamically, it can serve up different views of data for multiple use cases without the need to duplicate the data – otherwise you would need to deploy separate instances for every use case and that is expensive and extremely difficult to manage
With a trusted data foundation, you can develop models and scoring that use this data to make analytics and AI-based decisions.
Better value for you and your data teams
With the Quantexa Decision Intelligence Platform, you can create a trusted, joined-up view of your data. The platform provides your data as a service, which means it makes it available to your business in real time for use in multiple projects and initiatives.
Even better: Your data teams no longer have to spend so much time and manual effort bringing data together and dealing with data quality issues, and can instead divert their time to tasks that more meaningfully use their knowledge and skills. With Quantexa, they can rely on the accuracy of data and focus on extracting business-critical insights from it.
Ultimately, creating that trusted data foundation is the first step towards making your data operational. The right data management solution can be a game-changer—allowing organizations to derive value from their data, opening the doors to ambitious new AI and analytical initiatives, and delivering a true competitive advantage.
To find out more, download the report.
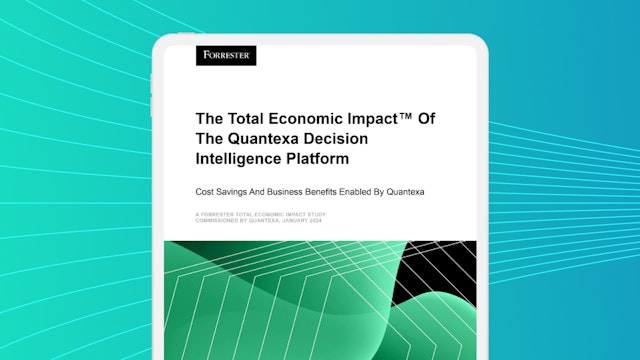