How Knowledge Graphs Give Generative AI Better Context
Understand which jobs require a knowledge graph, and when a vector database is the better tool.
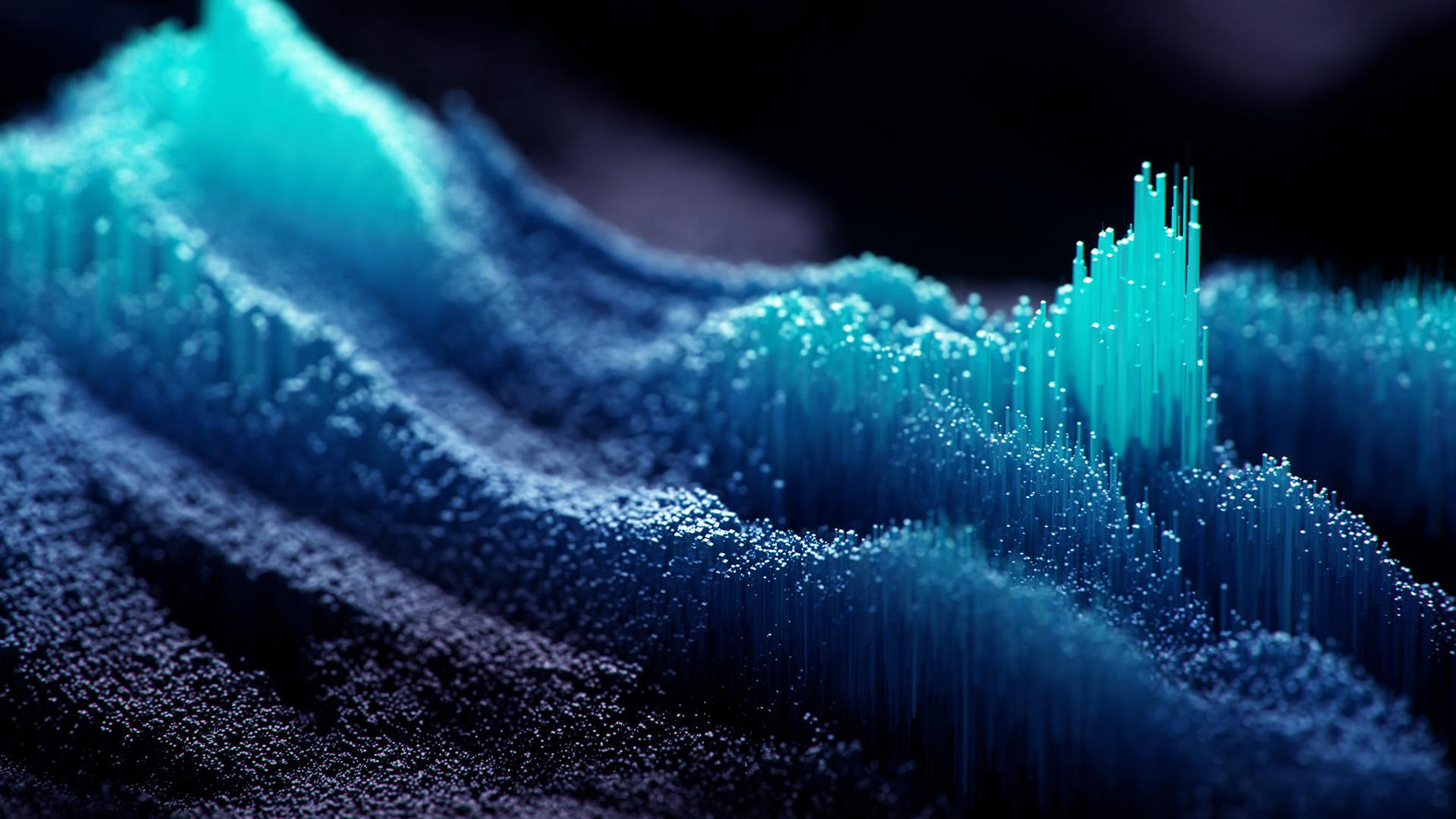
Large language models (LLMs) are useful in many cases but often seem like blunt instruments for a delicate job. LLMs can be fine-tuned, but it's an expensive endeavor. As a result, deploying enterprise context in conjunction with LLMs has become formalized in retrieval augmented generation (RAG) strategies or pipelines. Two such contextual vehicles are vector embeddings and knowledge graphs.
Of these, vector databases—searchable stores of vector embeddings, numerical representations of (often unstructured) data crafted to capture data’s semantic meaning within a high-dimensional vector space—have captured AI mindshare. Start-ups like Pinecone have been awarded huge valuations overnight.
However, some features of vector databases also carry overhead. For example:
An embeddings model that supports your preferred sentiment
Translation of data sources into a structured embeddings model; the larger the document or asset, the more costly and untimely the translation
Indexing and metadata handling
Efficient search
For use cases where knowledge is already known, vector databases can be computationally expensive instruments, like using a sledgehammer to crack a nut. Thus, knowledge graph advocates have made a strong case that their inherently knowledge-centric technology should be adopted—often standalone, but sometimes in tandem with a vector store.
In May 2023, a wonderfully creative post titled Generative AI is Graph’s Biggest Ever Opportunity asked GPT4 when it was best to apply knowledge graphs or a vector database. We have adapted some of the questions asked in this article to instead pose to Microsoft Copilot.
Prompt 1: You're given two stores of external information to choose from a knowledge graph or a vector database. Think about the steps involved in completing a question or task you (as an LLM) are given. Which of these two store types would be more likely to give you the text you need?
Microsoft Copilot: Certainly! Let’s explore the characteristics of both a knowledge graph and a vector database to determine which one would be more likely to provide the relevant text for a given question or task:
Knowledge Graph:
A knowledge graph is a structured representation of information using nodes (entities) and edges (relationships). Each node corresponds to a concept, and the edges denote connections between these concepts.
Structured: Knowledge graphs have a well-defined schema, allowing precise modeling of relationships.
Rich Context: Nodes can have attributes, and relationships carry specific meanings.
Reasoning: Knowledge graphs support inferencing and reasoning.
Use Cases:
Question Answering: Knowledge graphs directly provide answers based on their structured nature.
Entity Linking: They excel at linking entities across different domains.
Recommendation Systems: Graph-based recommendations leverage relationships.
Semantic Search: Graph-based search captures context and semantics….
Example: If you need information about the relationship between “Albert Einstein” and the “Theory of Relativity,” a knowledge graph would be helpful.
Vector Database:
A vector database stores data as high-dimensional vectors (numerical representations) in a vector space.
Embeddings: Each entity or concept is represented as a vector.
Similarity: Similar entities have similar vector representations.
Scalability: Vector databases handle large-scale data efficiently.
Use Cases:
Similarity Search: Vector similarity allows finding similar items (e.g., images, documents).
Recommendations: Vectors enable personalized recommendations.
Anomaly Detection: Detecting outliers based on vector distances.
Example: If you want to find similar documents based on their content, a vector database would be more suitable.
In summary, if the task involves structured relationships, context, and reasoning, a knowledge graph is preferable. For similarity-based tasks or scalability, a vector database would be more useful.
Prompt 2: Hallucination is a significant problem for LLMs such as yourself. If the information you need to perform a task is available to you in a knowledge graph, do you think this problem could be eliminated?
Microsoft Copilot: Hallucination refers to the phenomenon where a language model generates responses that are plausible-sounding but factually incorrect or invented... Let’s discuss how a knowledge graph might help mitigate this issue:
Structured Information:
A knowledge graph provides structured information with well-defined relationships. Each node (entity) and edge (relationship) has a specific meaning.
By relying on a knowledge graph, I can access factual information directly from the graph rather than generating it based on probabilities... The knowledge graph can supply the correct answer without relying on creative generation.
Reducing Ambiguity:
Hallucination often occurs due to ambiguity in natural language. A knowledge graph can disambiguate terms by linking them to specific entities.
For instance, if the query involves the term “Apple,” the knowledge graph can distinguish between the fruit and the technology company.
Reasoning and Context:
Knowledge graphs support reasoning and context-aware retrieval. If I need to infer information, the graph can guide me logically...
Fact Verification:
A knowledge graph allows fact-checking against reliable sources. If I encounter a dubious claim, I can verify it using the graph...
However, the complete elimination of hallucinations is challenging even with a knowledge graph:
Incompleteness: Knowledge graphs may not cover all possible facts or recent developments.
Contextual Nuances: Some queries require context beyond what the graph provides.
Creative Generation: Even with factual data, generating creative responses (e.g., poems, stories) involves additional reasoning.
The Microsoft Copilot responses are interesting. When determining your RAG approach, use case matters.
When knowledge of entities is at stake, knowledge graphs are better. Entity resolution and linking is the core raison d’être of the Quantexa Decision Intelligence Platform, and entity-to-entity networks underpin Quantexa Knowledge Graphs. Quantexa Knowledge Graphs, too, can incorporate entity information derived from unstructured data sources, for example, analyzing entity network information in conjunction with suspicious activity reports.
However, when your AI requires complex unstructured datasets, searchable vector databases are a great medium. Consider a bank determining pricing details for a fixed income portfolio of 100 OTC bonds, each bond with distinct non-standard terms embedded within PDF documents.
For some cases—for example, recommendations on films—known, networked (graphed) knowledge of a customer’s film type preferences in an online media entertainment system can overlap with a personalized similarity search. Both graph and vector approaches could apply.
On hallucinations, knowledge graphs provide clear, traceable “fact-checking” through logical guidance contained within the graph structure.
Microsoft Copilot warns of “incompleteness”—the graph only knows what it knows. However, when analyzing known entities, Quantexa Knowledge Graphs derive connections and facilitate analysis of wide-scale entity-to-entity relationships from your full structured and unstructured data estate. Data scientists can directly operate immediate, flexible analysis, such as PageRank algorithms, on the graph.
To be useful to the enterprise, LLMs must access external knowledge and data. Knowledge graphs offer a powerful repository of direct, traceable knowledge of entities and knowledge structures for RAG pipelines. Quantexa Knowledge Graphs offer insightful and interactive contextual entity-to-entity knowledge, which facilitates impactful knowledge engineering.
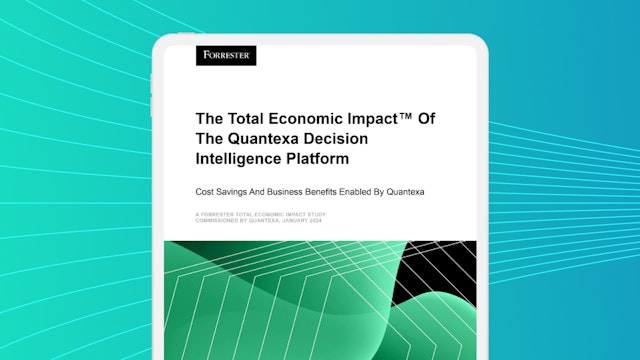