Risk Scoring and Intelligence Reports: Turning Data into Actionable Insights
Investigators have all the data they need, they just require the right technology to harness its true value.
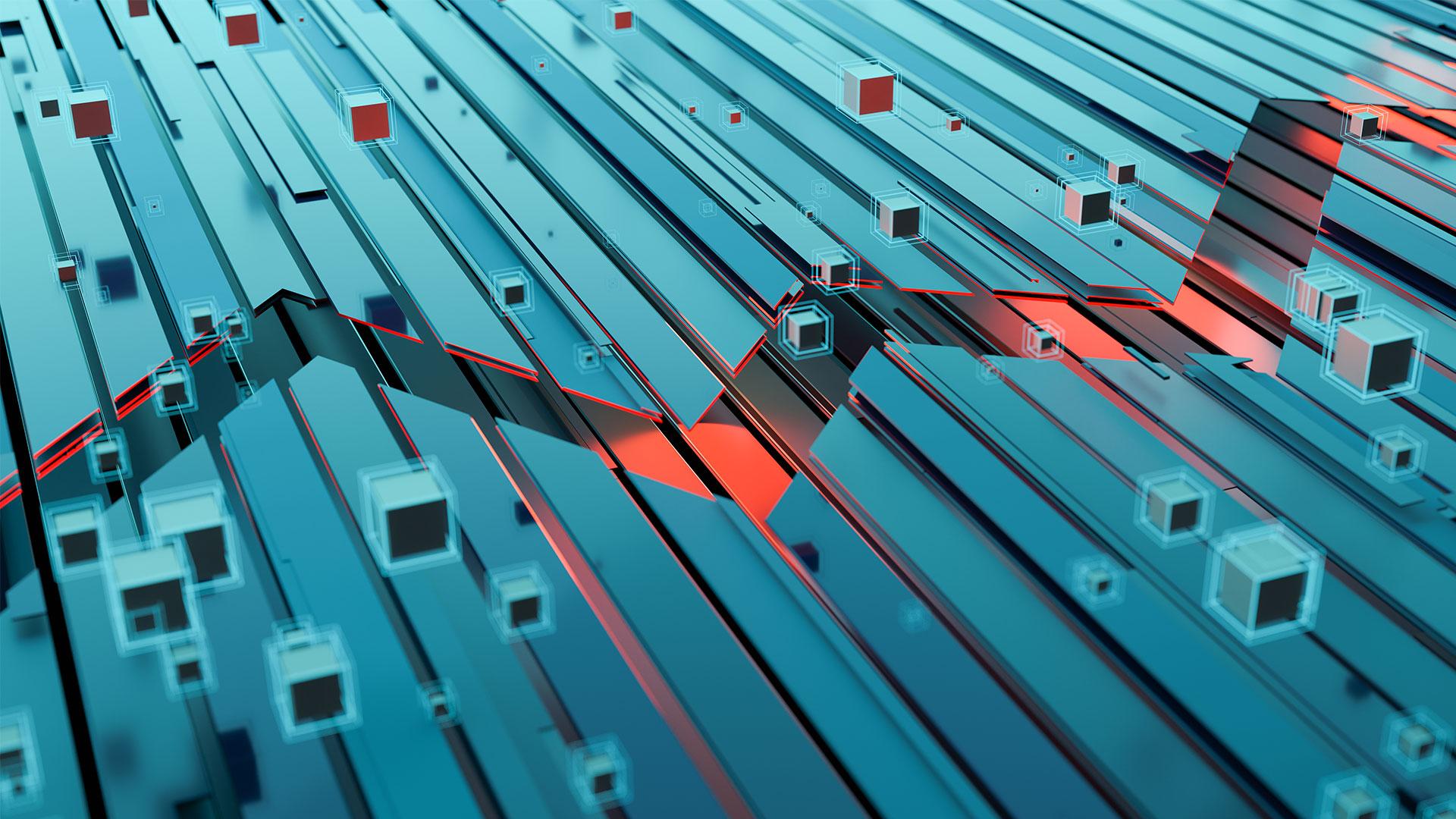
Estimates from the United Nations Office on Drugs and Crime (UNODC), the IMF, and the World Bank estimate that 3%-5% of global GDP — approximately $2.5- $5.5 trillion — is laundered annually worldwide. Transnational organized crime syndicates, narcotics traffickers, terrorist organizations, and kleptocrats are representative of the criminal entities trying to disguise illicit funds as legitimate transactions.
As these perpetrators’ skills at eluding detection evolve, so too must the methods employed by those tasked with catching and prosecuting them. In a changing landscape being driven by data and technology, money-laundering investigators are asking:
Who?
Identify actors (individuals, organizations), beneficial owners, profiles, associations, and risk factors such as PEP/watchlist status and past activity.What?
Detect suspicious indicators: unusual amounts/patterns, high-risk transaction types (gift-cards, crypto), questionable purposes, shell companies, evasion tactics (structuring), and abnormal account activity.Where?
Pinpoint prevalent locations: high-risk jurisdictions, virtual spaces (wallets/gaming), and physical locations (branches/ATMS) tied to illicit finance.When?
Analyze critical timing: unusual times/days, proximity to events, transaction sequencing, and frequency indicating coordinated activity.How?
Employ detection methods: rule-based systems, anomaly detection, link analysis, behavioral analytics, entity resolution, NLP, data mining/ML, human intelligence, and information sharing platforms.
Key indicators of illicit transactions
Money laundering is a complex financial crime that often involves a series of transactions designed to obscure the origin or source of funds. But not everyone who exceeds a transaction threshold or turns up on a money transfer form is necessarily doing illicit things. So, how can the bad actors be identified?
While there's no single definitive pattern, analysts look for a combination of indicators and suspicious behaviors that may include:
Unusual transactions: Frequent, large cash transactions, structured transactions to avoid reporting thresholds, or sudden increases in account activity.
Complex structures: Involving multiple accounts, jurisdictions, or financial institutions to obscure the source of funds.
Suspicious activity: Transactions involving high-risk jurisdictions, illicit industries, or known criminal organizations/contacts.
Information discrepancies: Inconsistencies between the stated purpose of a transaction and the actual nature of the funds. Additional intentional misrepresentation due to name or address variations is also common.
Applying a risk-based approach
To identify potential money-laundering activities, government agencies rely heavily on the use of the Bank Secrecy Act (BSA) data collected by the Financial Crimes Enforcement Network (FinCEN). More than 260,000 regulated financial institutions submit over 55,000 reports daily (+20 million annually) to FinCEN, and the data is used by law enforcement, regulatory bodies, and national security agencies to combat money laundering, terrorist financing, and other illicit financial activities.
These agencies often employ a risk-based approach that considers factors such as:
Geographic components: Transactions involving distant locations (e.g., 50 miles) from the account holder's residence or business. Multiple transactions at different locations in a small-concentrated geographic area over a short period. Flows from counties or regions that are known for high risk.
Transaction frequency: Frequent (burst) or unusual patterns of transactions. Multiple days in a row, different times of the day, or across different financial institutions on the same day/week.
Similarity: Shared email or IP addresses across multiple accounts (money mules) or transactions. Use of a common address (safe house). The reuse of alien registration or passport numbers in different areas.
Data variations: Inconsistencies or patterns in reported dates of birth, ID numbers, and addresses. Frequent use of character or number transpositions, typos, and misspellings.
Occupation indicators: Certain occupations may be associated with higher risk (e.g. unemployed, student, pilot, massage therapist, doctor, executive, police officer, housewife, etc).
Narrative keywords: Specific keywords in transaction descriptions and narratives may indicate drug dealing, weapons trafficking, human smuggling, or terrorism. For example, words such as: structuring, layering, avoiding, hiding, smuggling, overseas, extremist, violent, confused, uncommon, questionable, etc.
Account relationships: Connections between different accounts, particularly those with unusual activity such as high-velocity transfers, the direction and flow of funds to a single account, or a large fan-out or usage of different accounts.
Trying to distinguish these complex factors, however, would be impossible without today’s high-tech solutions. This is why savvy investigators are turning to advanced analytics to help prioritize high-risk subjects to allow financial institutions and government agencies to consistently and reliably detect these situations.
The diagram below presents a comprehensive analytical model that incorporates various Bank Secrecy Act (BSA) forms (SAR, CTR, CMIR, FBAR, 8300, MSBR, DEOP, and BOI) and their associated entities and interconnections. Each transaction entity provides detailed information, including timestamps, amounts, filers, locations, and information about involved parties (suspects, individuals, and businesses).
Image: A representative analytical model for BSA
Entity resolution is the foundation for better decision-making
By minimizing inconsistencies and duplications in entity data, analysts can make more informed decisions to help them “follow the money.” Entity Resolution plays a critical role in integrating the data to ensure a consolidated and reliable view of the information.
How bad actors are playing the system
One common red flag in BSA data is the use of the same Social Security Number (SSN) by multiple individuals. Assuming the SSN is not an invalid number like 999-99-9999, 000-00-0000, or a variant thereof, it would be highly unusual to encounter this when dealing with legitimate financial transactions.
While transcription errors can occur, particularly in isolated cases (2s sometimes look like 5s, 4s like 9s, 3s like 8s, and 7s like 1s), the repeated use of an SSN by different individuals is highly suspicious. This pattern is illustrated in the image below and often indicates potential identity theft, fraud, or other illicit activities.
Image: Multiple subjects using the same SSN
Conversely, another intriguing pattern emerges when a single individual uses multiple SSNs. While entity resolution typically addresses common duplication issues, instances where a person is associated with multiple, distinct SSNs warrant further investigation, as this anomaly could indicate potential identity fraud, intentional misrepresentation, or other underlying reasons. The image below illustrates this pattern.
Image: Single subject connected to multiple SSNs
Another interesting BSA pattern involves the identification of "safe houses" where different individuals (unrelated to each other) use the same address for their financial transactions.
While it's not uncommon for family members to share a residence, a pattern of multiple individuals, especially those who are unrelated, using the same address for frequent and suspicious transactions is highly indicative of potential money laundering or other illicit activities.
The pattern shown in the image below is structurally similar to the previous patterns, but it carries a different connotation and interpretation that often suggests the involvement of money mules in schemes such as elderly abuse, romance scams, or other fraudulent operations.
Image: Safehouse used by multiple subjects
Finding a single view of perpetrators with decision intelligence
Quantexa’s Decision Intelligence Platform and Financial Crime solutions are designed to identify and encode these patterns, generating dynamic risk scores based on the selected data. This enables task-force members to establish geographical targeting orders (GTOs) to detect specific behaviors, SAR review teams to monitor jurisdictions for criminal activity, and government agencies to match overlapping targets with ongoing investigations.
Analysts receive a well-vetted list of suspects, ranked by risk score and accompanied by detailed information on the applicable patterns. This approach establishes a robust baseline for investigating money-laundering activities, leading to more comprehensive intelligence reports (including seizure warrants or congressional inquiries) that drive investigations, increased asset forfeiture, and higher conviction rates.
Investigators have all the data, they simply need the right technology to harness its value by connecting billions of data points that may be spread across multiple systems into a single, accurate view that reflects the real-world connections between people, places, and organizations to stop today’s sophisticated money launderers.
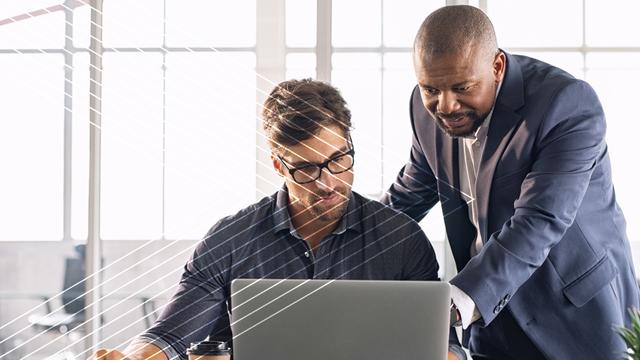