Contextual Monitoring: The New Technology Retail Banks Are Using to Stop Money Laundering
We know traditional transaction monitoring doesn't work, but how can banks proactively identify risk by following the money? Our experts show you a more efficient and effective approach.
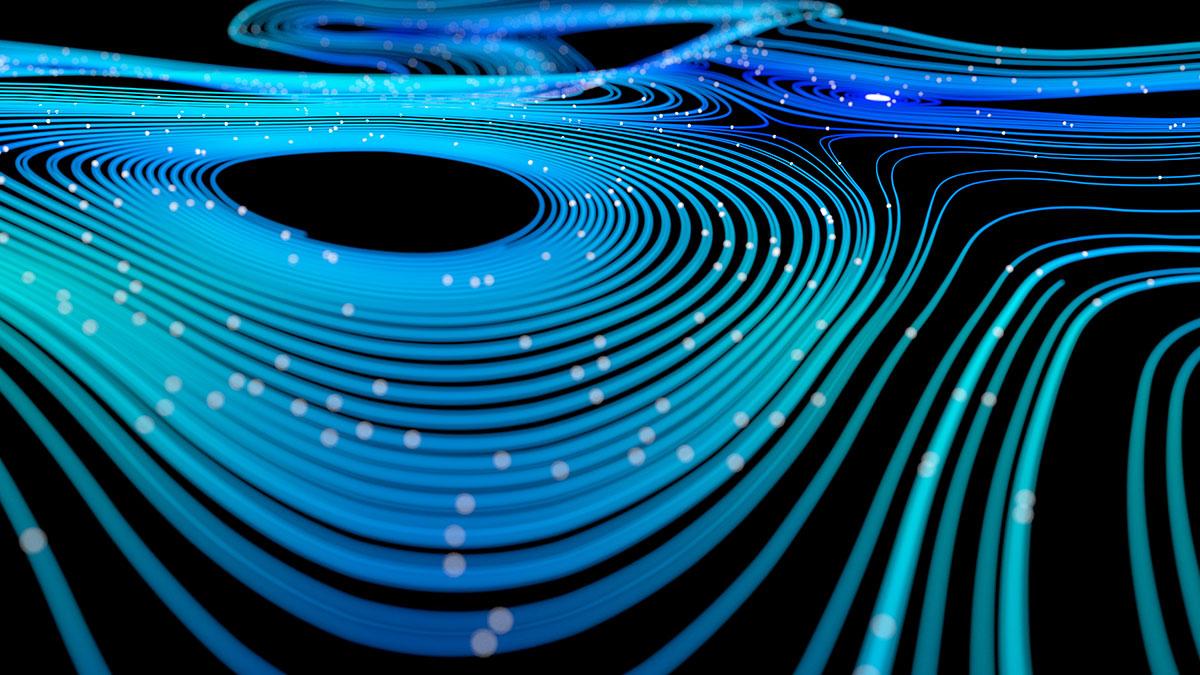
The Nasdaq Verafin 2024 Global Financial Crime Report shows that “an estimated $3.1 trillion in illicit funds flowed through the global financial system in 2023.” The report goes on to say that, “money laundering accounted for trillions of dollars funding a range of destructive crimes, including an estimated $346.7 billion in human trafficking and $782.9 billion in drug trafficking activity, as well as $11.5 billion in terrorist financing.”
None of this is news to financial crime compliance officers, of course, who are all too aware of the battles being waged against money launderers every day within their organizations—a task made even tougher by budget cuts and staff reductions.
So, how can banks do a better job of curbing these illicit activities that are getting more complex and difficult to detect with outdated methods?
To answer this question, we need to start by examining how financial institutions view risks today, the limitations on traditional ways of looking at risk, and what the benefits are of moving beyond traditional transaction monitoring to a risk-led, contextual approach that utilizes the latest technology to provide a far more holistic view of customers and their counterparties.
Isolated alerts tell part of the story, but not the whole story
Most financial crime compliance officers believe they have a comprehensive profile of their customers and with good reason. With KYC onboarding controls, customer risk assessments, and AML monitoring in place, there is a level of monitoring taking place throughout the customer's lifecycle, and if a number of transactions made by an individual look suspicious, financial crime units can follow up.
The problem with traditional transaction offerings like this, however, is that they look at a customer's transactional activity in isolation, relying on value/volume-based controls within the bank’s network. While valuable, this is only one piece of the puzzle.
Seeing outside of the bank’s network to get the full view of customers and their counterparty connections is the only way to determine that these aren’t lone actors but rather players in a far wider criminal network that may be laundering money through the bank for any number of nefarious purposes.
“Following the money” this way also transitions money-laundering investigations away from traditional reactive methods to a proactive way of looking at larger clusters of transactions that identify broader criminal networks. There are also several practical reasons for moving beyond transaction monitoring.
Why single red flags are a roadblock to finding criminal networks
Tracking suspicious transactions based on a single individual requires huge investigative teams, and these teams are costly to operate. More importantly, following single “red flags” greatly increases false positives.
Investigating 10,000 false positives a month squanders valuable investigative time, drives costs, and simply isn’t an efficient way to follow the money. Banks may also find it hard to attract and retain good analytic talent if all they’re being asked to do every day is play Whac-A-Mole to verify thousands of alerts when the real risks are being missed.
The even larger question here, however, is: how can the industry or regulators realistically expect an AML ops analyst to uncover an organized criminal network based on a single red flag in a transaction?
Moreover, the controlling mind behind an organized criminal network might very well sit within a bank’s retail book. Without the tools to identify the connections to this entity from inside and outside of the bank’s network, it is impossible to uncover the full web of risk associated with that individual.
To move to a more optimal solution, analysts need to greatly curtail the number of false positives they're chasing. Seen in this new light we might ask: what if you could reduce those 80% false positive rates down to something more manageable like 20 to 30%? There is a way, and it starts by looking for multiple red flags revolving around a single entity instead of chasing single transactions.
Having the ability to look both inside and outside of the bank’s network opens the door for investigators to identify multiple red flags or “clusters” of transactions at a network level and aggregate risk to a scorecard that moves far beyond a single transaction to begin to answer whether the money came from a questionable source and, most importantly, where it went.
Looking at the entire network of risk in this manner and having the ability to see customers, their counterparties, and "clusters of risk" is now possible with Quantexa's Contextual Monitoring.
Why transitioning to Contextual Monitoring makes sense
Contextual Monitoring is a true paradigm shift in how transaction monitoring is conducted. It provides a clear roadmap to dramatically improve financial crime monitoring and investigation controls and processes by providing context to the data.
This solution maintains transactional risk analysis inside a bank’s network, but it extends the overall view of risk outward to provide to a full 360-degree view of customers, linking customers and their counterparties together to show the true network relationships that exist—all in real-time.
This added context helps to identify hidden risks and generates more accurate alerts so that investigative teams chase fewer false positives. It also empowers analysts to look beyond the payment itself to understand the broader context of the parties involved and, therefore, whether there is risk truly present.
Underpinned by Entity Resolution and Graph Analytics techniques powered by AI and machine learning, Contextual Monitoring focuses on holistic relationships rather than individual transaction values and volumes.
Quantexa's Decision Intelligence Platform is a revolutionary technology powering a Contextual Monitoring capability that is designed to ingest massive volumes of data. As a result, it can automatically consolidate and connect internal and external data for a complete view of customers and their counterparties. This uncovers hidden risks quickly and more accurately, accelerating efficiencies and effectiveness. Sophisticated visualization models and an intuitive UI then help to focus investigative actions on real risk.
Here's how it works:
Using Contextual Monitoring to follow the money
Supervised machine learning (also known as "predictive") uses historic cases to statistically identify patterns. However, certain use cases tied to predicate criminal offenses may have very few previously known examples. The following journey can be used when predicting risk for these use cases:
Quantexa has established typologies for several predicate criminal offenses tied to human trafficking, terrorism, modern slavery, shell companies, and more. Based on indicators and guidance that have been published by various regulators around the world, Quantexa has used these characteristics as a baseline to establish "scorecards" for various types of suspicious activity and behavior. With these specific combinations of granular scoring, Quantexa's scorecards clearly point to activity that is indicative of these identified offenses.
For example, let's say you have your monitoring system set up to flag any transaction above $50,000, which could be a change in behavior and far above the transactional amount for a normal customer. If that money came from an escrow account, you might not flag it as being suspicious because you're only seeing it as money being used for the purchase of a home. But by using the Contextual Monitoring approach, you can see a much wider and enriched view of the customer associated with this transaction to fully expose any potential risk from what funded the escrow and any potential risk associated with the property such as where it is located and the number of times the home was bought and sold in the past 12 months.
Now you can see all of the additional data points linked to a customer's activity to identify whether this is someone who is in a high-risk geography or is known as an associate through negative news or if it is an entity that is linked to someone on a terrorist watch list. Suddenly, you're able to expose that risk and elevate that alert because you have the additional context via their connections to what that risk is.
Trying to use an open public search for this kind of information manually is an all-consuming task that takes precious time away from analyzing information. If you're employing a platform that does all of the data enrichment up front, however, you're saving time and resources. Most importantly, you're engaging your analysts in what they like to do best—find the real risks that are being missed and follow the money.
Financial crime compliance made easy with Contextual Monitoring
Effective monitoring to curtail money laundering starts by having a solid foundation of quality data. Once that foundation is established, analysts can then begin to look for the answers and clues hidden in that enriched data pool to determine:
Who is the person or entity that the money came from?
What did they do with the funds?
Where did the money go?
Who is the person or entity that the money went to?
Does the activity make sense or appear tied to a criminal offense?
Automating these steps in the investigation process is what Contextual Monitoring does to build that solid data foundation from which enterprises can drive more thorough analyses and, ultimately, better decisions.
The key benefits of taking this approach are:
Risk profiling non-bank customers and their counterparties in context is a massively growing regulatory expectation. In our next blog post, we'll show you how Contextual Monitoring allows investigative teams to laser focus on clusters of risk and networks to more easily illustrate to regulators how they are curtailing financial crimes.
To learn more about how Contextual Monitoring can help your organization, click here.
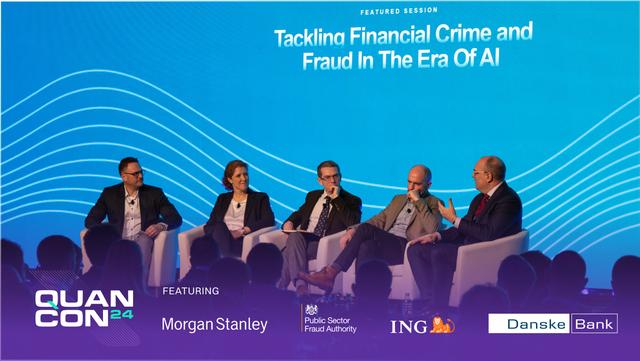