4 Ways to Fix Poor-Quality Data for Good
Stop running from chronic data quality issues and bring your data—and your business—under control.
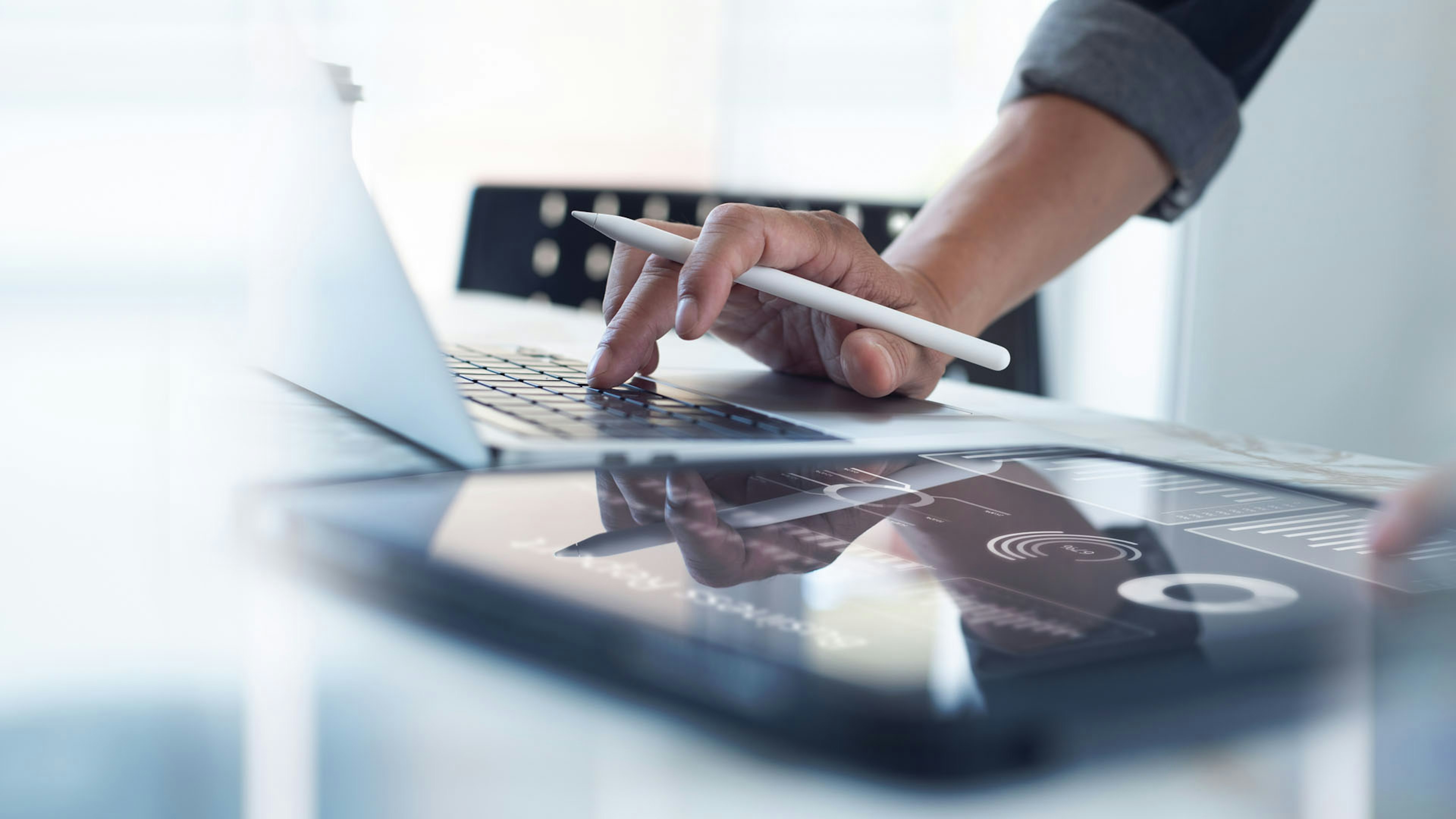
Data quality issues are nothing if not persistent. Some data specialists see the ultimate fix as validating data when you first enter it into your business systems. If only it were that simple.
One of the major obstacles in improving data quality is that the task can feel endless and overwhelming. Imagine information being sorted and organized by many different people, each with their own approach. Sometimes, data is entered inaccurately or in a way that leads to degradation or even loss. And sometimes, important data is simply missing. On top of this, new data is constantly flowing into the system.
Data management can seem like a Sisyphean task. And even once your data is resolved, normal human behavior intervenes to unravel your good work: End-users both intentionally and unintentionally foil the best intentions of system designers. Users who take shortcuts don’t realize the impact their actions might have on your downstream data consumers.
Over time, and across multiple systems, these errors compound, creating complexity and becoming constraining—not to mention costly—for your business.
How the cycle of poor data quality begins
Data quality problems often start at entry points, like online forms or customer data inputs entered by operations teams. Each point gathers different details about an individual. But the risk of human error persists (think: misspelled names or mistyped information).
Validation points, such as address lookup or prompts in a free-text field, help ensure that information is entered correctly. However, even then, it’s only natural for users to look for shortcuts, despite the effect that workarounds can have on upstream data use.
It’s typical to find dummy text entered in mandatory fields to bypass the system, even from trained operations staff. And when you finally bring the data together from multiple customer systems, you realize just how out of sync it is.
Data quality management requires a new approach
Traditional approaches to improving data quality aren’t effective. A critical step is connecting data from different sources to have a trusted, deduplicated view. However, record-to-record matching,that is used by most tools., struggles to deal with sparse, inaccurate data and is ill-suited to natural variations in data sources. And even if a single view is achieved, it is not flexible to adjust to user access restrictions around sensitive data sources, in which different use cases have different requirements. Too often, replication is the way traditional approaches deal with different use cases—which, in a large organization, can quickly become unmanageable.
Plus, traditional approaches do not take advantage of context. The process of data quality management normally involves assessing data source quality in isolation. More advanced methods allow cross-enrichment across data sources, meaning you leverage context coming from all your data and therefore get far more accurate results.
Also, to connect data many traditional systems require a fixed schema to ingest the data which can be a very lengthy process, slowing down time to value and as a result, reducing the number of data sources used, missing out on value.
Differing use cases and sparse, incorrect data are just some of the many reasons that data quality management remains such a challenge: Poor data quality stemming from duplicated records, missing information, and even intentional manipulation by criminals can further hinder the process, as can unintentional siloing of data across internal and external systems, resulting in a disjointed and incomplete picture.
As more and more organizational decisions are made on the back of data, enterprise leaders are in dire need of powerful solutions to help solve chronic data quality issues once and for all.
AI-powered solutions are supporting better data quality
There are technologies on the market that can address data quality challenges, for example, Quantexa’s Decision Intelligence Platform, which offers you several ways to work through your data quality issues (instead of constantly working around them):
1. You can connect all of your data into a single view
Unification of data is a critical step toward improving data quality. Quantexa lets you cross-enrich sources so you can gain a far more comprehensive picture of your data. The platform is built on best-in-class Entity Resolution. So, you can build a single view of your customer, making connections even when individual source records are incomplete.
2. You can create candidate data profiles based on a single view
By using rules in Quantexa, you can create a candidate record that contains all the attributes, scores the data, and enables your teams to make decisions about a particular record. And when you link records from the start, you avoid creating duplicate records, which can undermine overall data quality and dilute your efforts to create a single source of truth.
3. You can evaluate your data for quality issues
Obviously, if you want to improve data quality, you need to be able to look for quality issues with speed and ease. With Quantexa, you can decide on the most reliable attributes to create a trusted record. By scoring your data against these attributes, and using Quantexa’s analytics framework and data exploration features, you can quickly locate conflicts in the data and automatically handle those issues. Or, if they are severe enough, you can flag them for human interaction.
4. You can create a continuous process for improving data quality
When you can conduct quality scoring on the single view that Quantexa provides, you are empowered to continuously monitor and improve your data quality. With this focus on improvement, the amount of serious data quality issues will subside over time, so only minor issues remain.
Improved data quality mitigates risks from regulatory challenges
Resolving poor data quality issues creates many business benefits—and chief among them is the reduced risk of facing arduous and costly data remediation efforts to satisfy regulators.
Regulatory drivers enforce major rectification exercises to ensure companies have a more accurate view of their finances and control over their business. For example, in the banking and finance industries, companies must comply with Know Your Customer (KYC) regulations and be able to define the legal entities that make up their multinational customers.
The regulatory factors push companies into compliance by having audit points they must meet. Complying with these regulations comes at a significant cost when you have several teams manually trying to reconcile records. And if you wait until the last minute to reconcile your data, it’s even more costly because of the extra resources and work required to meet the deadline.
When data quality is improved, everyone benefits
Because the Quantexa Decision Intelligence Platform helps you to work through and solve poor data quality issues, those problems won’t get in the way of meeting compliance requirements. Automation also helps you reduce manual work—and costs—associated with data quality management. And with more control and continuous improvement over your data, you can work simultaneously on new data projects that serve your customers’ needs and drive your business forward.
In short, when you use Quantexa to improve data quality, you can improve your data’s impact on your business—and ultimately, the impact of your business on your customers.
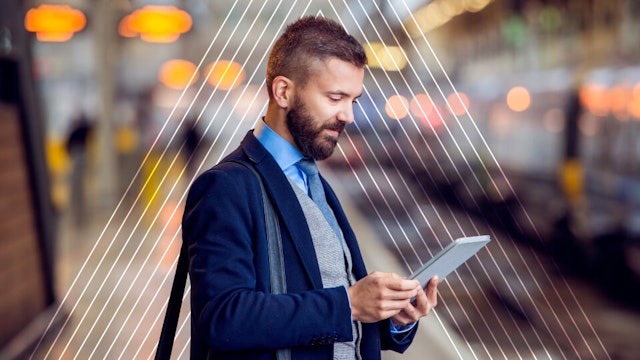